Premise: Digital business differentially uses data to create and sustain customers. In a digital business, data is an asset that must be valued, invested in, and properly managed.
The crucial role of data in organizations has been studied extensively. Entire industries have evolved structures to handle problems like information asymmetries, economic opportunism, and risk sharing. Moreover, new research from cognitive science and behavioral economics are reshaping the notion of “rational actor” in markets, pushing businesses to focus on factors like customer experience as a proxy for more concrete measures of customer choice. Finally, the emergence of big data as a set of disciplines for gleaning deep insights from seemingly unrelated data sources is having a profound effect on business behaviors, from executive decision making to automating responses to customers.
But there’s little data on one aspect of data: Value. For digital business decision makers, the lack of a reasonable framework for assessing data value is problematic: Without a framework for assessing data value, digital investments will almost certainly be sub-optimal. We know that data must be valuable, but to agree on how it to value it, we must adopt a number of concepts, including:
- Data value is based on use. From a financial perspective, data has limited value because it is so easy to copy, share, and reuse. But from a digital business perspective, data has significant value: It’s the basis for differentiating a digital business. A different view of the value of data is required, and that view is that data should be valued based on how it is used.
- Data, in context, is capital. Although data doesn’t easily show up in financial statements, that doesn’t mean it isn’t crucial to generating business outcomes. Data must be placed on an equal footing with other productive assets, like machines, labor, and money, to make sense of a digital business.
- A framework for managing data as an asset. If data is capital, then we should be able to describe it using capital-related concepts like “income” and “retained earnings.” We can, and it leads to a simple framework for managing data value.
Data Value Is Based on Use
Adam Smith observed that there are two types of value: value in exchange and value in use. Value in exchange is based on scarcity and is largely time independent; value in use is based on utility and is highly dependent on the moment. To show the difference between the two, Smith used diamonds and water as examples. Diamonds (in 1776) had little value in use, but because they were scarce, cost a lot in exchange. Water, by contrast, had little value in exchange (again, in 1776), but great value in use. To this day, most assets — including data assets — are valued based on their value in exchange: what would this asset cost to replace, or insure, or buy on the open market? For assets that follow the rules of scarcity — like a machine (operate in one or a few lines at a time), or labor (time is perishable), or cash (buy this, but not that) — value-in-exchange frameworks work just fine.
The problem is that it’s hard to measure an intrinsic value-in-exchange of data because data doesn’t adhere to the rules of scarcity. Data can be used by you and me at the same time, without any loss of fidelity. Of course, some data-oriented industries have erected significant legal codes to turn data into something scarce. Publishing, for example, uses copyright law to assign ownership to data and guide commercial behavior for data products. But the dominant economic feature of data is that it is easily copied, edited, shared, and reused. The same data might be used by two different people at the same time, or the same person at two different times, and have very different productive impacts. The simple truth of data is that its value is best determined in terms of its use.
Data, In Context, Is Capital
Data may best be valued according to its use, but that doesn’t mean we can’t coherently describe core attributes of data value. Evaluating the value of data in use is possible with four concepts. The first data value concept is that data value is context dependent: although data can be readily reused, that doesn’t mean that its value is the same in all uses, at all times. The second is that data is capital: despite not following the rules of scarcity, data is a non-financial, formatted asset that is essential to the production of goods and services. The third is that data can amplify its value: any business activity generates derivative data that itself can be greatly valuable to the enterprise. The final concern is that data is costly to retain; choices regarding what data, and in what form, to retain and where to retain it are among the most important digital business decisions a manager can make.
A Framework for Managing Data As An Asset
Wikibon has developed a simple model for understanding the relationships between context, data capital, data income and retained data value (see Figure 1). It’s starts with the concept of a “business moment” during which a particular activity is performed in context, like a sales call, or a shipment, or a product roadmap decision. Almost every business moment consumes labor, machinery, money, materials — and data capital. Successfully performing the business moment has a number of effects, including throwing off cash, profit, a deliverable, customer satisfaction — and data income, which is the increase in data value above the value of the data capital employed. Data income is always positive. Why? Because data capital isn’t diminished by use; it can be shared across application and time. However, just because data income is always positive doesn’t mean that the business always can appropriate that income. Remember, data is expensive to maintain. Deciding which data to retain, and in what form, is crucial to managing data value. Do you maintain raw data? Or just the data in “insight” or “model” form? Or do you just let the data dissipate, like exhaust? The processes for retaining and formatting data income back into a portfolio of data capital is among the most important activity sets performed by a CIO or CDO — or whoever is entrusted to maximize data value in the enterprise.
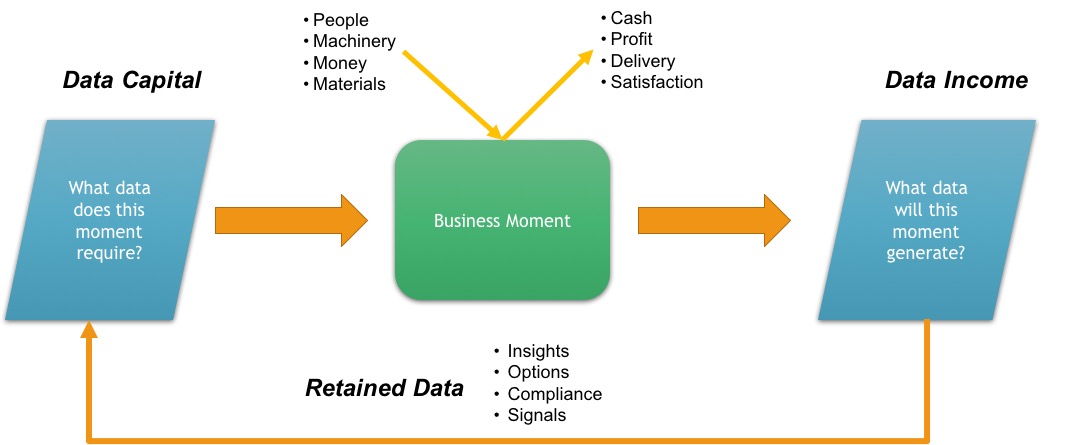
Tools for managing data as an asset take many forms, but most, like data dictionaries or data lifecycle management tools, are rather specialized, built to serve a particular audience, and don’t support concepts like data retention. Tools for cataloging data assets are emerging out of the big data ecosystem from established vendors (Microsoft), start-ups like Waterline Data and Alation, and open source projects like CKAN and DKAN. These tools can simplify data discovery, data wrangling, and other data preparation activities. However, while most data catalog tools provide a means to describe data resources, they don’t yet provide facilities for mapping networks of data, which will emerge as crucial to understanding how data capital turns into data income and, when retained, generates additional data capital.
Action Item: Efforts to intrinsically value data so that it can be demarcated on a balance sheet are not working, and probably never will work. Nonetheless, CIOs (or anyone responsible for monetizing data) need to establish basic frameworks for valuing data that can lead to proper digital business investment, architecture, and operations.