With George Gilbert
In the next 3-5 years, we believe a set of intelligent data apps will emerge requiring a new type of modern data platform to support them. We refer to this as a sixth data platform. In our previous research we’ve used the metaphor “Uber for everyone” to describe this vision; meaning software-based systems that enable a digital representation of a business. In this model, various data elements, such as people, places and things, are ingested, made coherent and combined to take action in real time. Rather than requiring thousands of engineers to build this system, we’ve said this capability will come in the form of commercial off the shelf software.
In this Breaking Analysis, we explore the above premise and welcome Ryan Blue to this, our 201st episode. Ryan is the co-creator and PMC chair of Apache Iceberg. He is a co-founder & the CEO of Tabular, a universal open table store that connects to any compute layer, built by the creators of Iceberg.
[Note: We recognize that there are other formats such as Hudi and Delta that will contribute to future data platforms. For this Breaking Analysis our main focus is on Iceberg and its disruptive potential].
Figure 1: The five modern data platforms and the products that comprise their systems along with some examples of contributors to the sixth data platform.
*AWS and emerging modular platforms are today largely bespoke products and tools that have the potential to become platforms.
Figure 2: ETR’s Technology Spending Intentions Survey shows spending momentum and market presence for the major data platform vendors. X axis represents account penetration. Y axis represents spending momentum. The graphic above shows spending data within the cloud for the leading data platforms. This specific cut of the data is for database and data warehouse offerings filtered by cloud customers. We include Oracle in deference to the database king, which continues to aggressively invest adding capabilities to its data platforms. It also provides relative context because Oracle has a sizable presence and is mature. The Y axis shows Net Score or spending momentum. It is a measure of the net percent of customers in the survey (N=1,165 cloud customers) spending more on a specific vendor platform. The X axis labeled as Overlap is an indicator of presence in the data set and is determined by the N’s associated with a specific vendor, divided by the total N of the sector. Think of the horizontal axis as a proxy for market presence. The red dotted line at 40% is an indicator of highly elevated spending velocity on a platform. The following key points are notable:
Figure 3: ETR’s Emerging Technology Survey (ETS). X axis is mindshare. Y axis is net sentiment.
Another capability of ETR’s data set is seen above from the ETS survey which measures the sentiment and mindshare for non-public companies. In the graphic above we chose to highlight four contributing players to the sixth data platform including Fivetran, dbt Labs, Starburst and Atscale.
The squiggly line at Starburst shows the positive progress since November of 2020. Note that each of these four would show a similar trajectory which is one reason why we chose to highlight them.
Figure 4: Key questions Ryan Blue of Tabular addresses about multi-vendor data platforms with open storage. In the ever-evolving world of data platforms, we saw the journey from the atomic and fragmented Hadoop ecosystem to the highly integrated systems of today, exemplified by Snowflake. We see a future of more pivotal shifts. Recent trends raise pivotal questions about usability, cost, and value in the data landscape.
Ryan:
Dave:
George:
George:
The above graphic is an attempt to illustrate what we see unfolding in the market that Ryan is helping us articulate. As we start on the left and we had a device or data platform-centric view of the world where it might be Redshift or Snowflake, where the state of the art of technology required us to integrate all the pieces, to provide the simplicity and performance the customers needed.
But as technology matures, we can start to modularized and the first thing we’re modularizing is storage. Now it means we can open up and offer a standardized interface to tables, whether it’s Iceberg or Delta tables or Hudi. And what Ryan is helping us articulate is that permissions have to go along with that. And there’s some amount of transaction support in there.
And then we’re taking apart the components that were in an integrated DBMS. Now, it doesn’t mean you’re going to necessarily get all the components from different vendors, but let’s just go through them. There’s a control plane that that orchestrates the work today. We know these as dbt or Dagster, Airflow or Prefect. There’s the query optimizer, which is the hardest thing to do where you can just say what you want and it figures out how to get it.
And that is also part of Snowflake and Azure synapse of Fabric or Databricks, which built their own Databricks SQL, separate from the Spark execution engine. The execution engine is an SDK sort of non SQL way of, of getting out the data. That was the original Spark engine. Snowflake has an API now, but we believe it goes through their query optimizer.
And there’s the metadata layer which goes beyond just the technical metadata. And this is what we were talking about with Ryan, which is how do you essentially describe your data estate and all the information you need to know about how it hangs together? And that’s like AtScale with its semantic layer, there’s Atlan, Alation, Collibra which are sort of user and administrator catalogs.
But the point of all this is to say that we’re beginning to unbundle what was once in the DBMS. That’s just the way Snowflake unbundled what was once Oracle, which had compute and storage together. They separated compute from storage and now we’re separating compute into multiple things to Ryan’s point. So that we can use potentially use different tools for different workloads and that they all work on one shared data estate that the data is not embedded in and trapped inside one platform or engine.
TL;DR
The Evolution of Open Storage Management and Its Impact on Modern Data Platforms
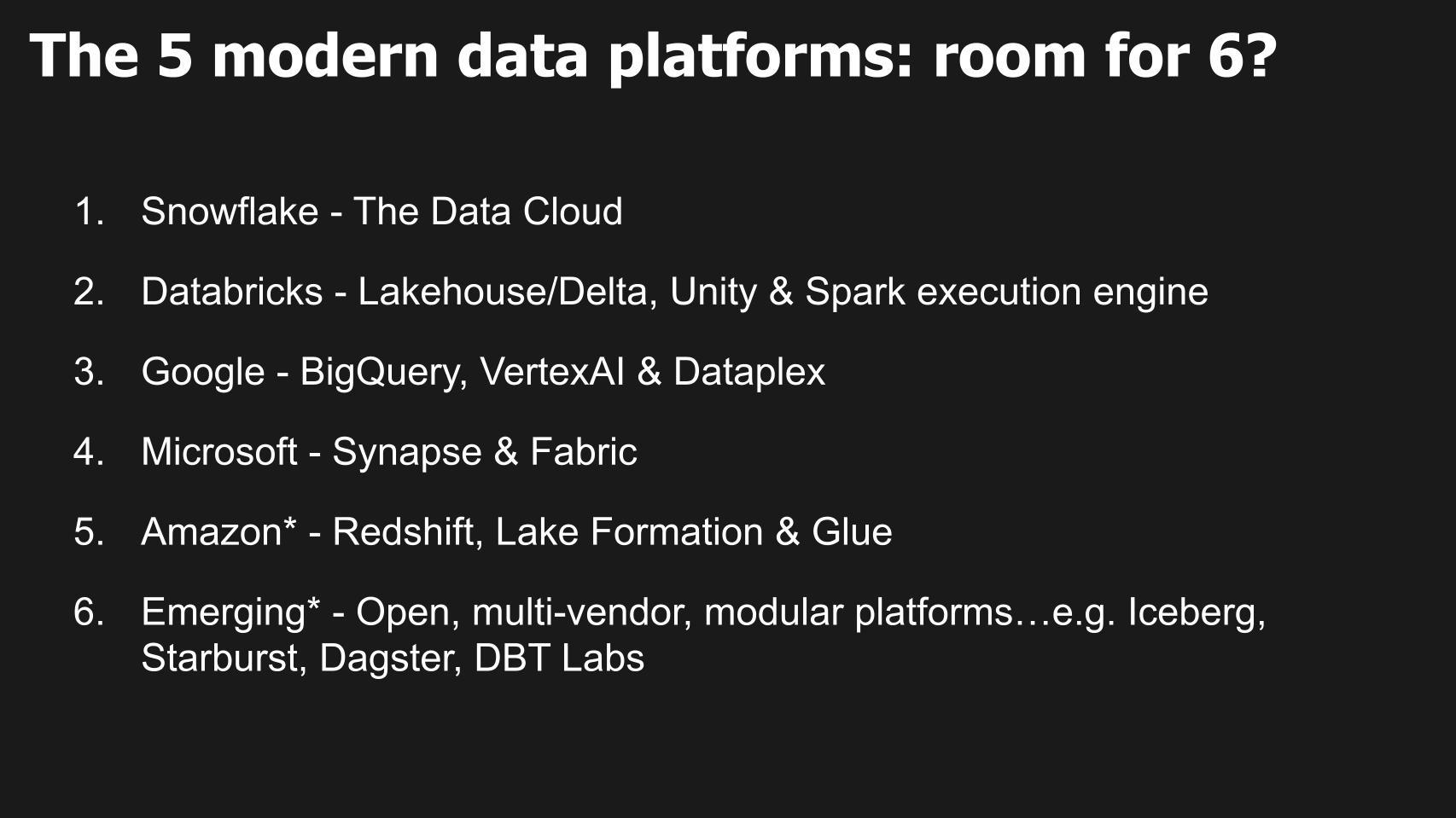
Introduction
Open storage managers, like Tabular and its use of Iceberg, in combination with other tools and products, have the potential to revolutionize the architecture and business models of existing data platforms. We use the term “platform” to represent an architecture comprising multiple products and tools that work in combination. Platforms have more comprehensive problem solving objectives than individual products and are more extensible in a variety of dimensions (see this more detailed description by Blaise Hale).Traditional Data Platforms Create Lock-in by Owning the Data
Historically, data platforms control customer data. Even though storage and compute may be architecturally distinct, they are effectively integrated. This integration means that owning the data gives platform vendors control over various workloads. This control spans business intelligence, data engineering, and machine learning due to the concept of data gravity and the need to share via pipelines.The Shift Towards Open Data Storage Increases Competition
Independent management of data storage and permissions gives customers more optionality and forces vendors to compete for every workload based on the business value delivered, irrespective of lock-in.Data Sharing Redefined
Data sharing within a single enterprise in the future necessitates the ability to read and write a single instance of the data using an open storage format. This forces multi-vendor standards for data sharing between enterprises.Examples of Players of the Emerging Modern Data Platform & Future Competitive Landscape
- Snowflake might excel as the most performant business intelligence service.
- dbt Labs could manage data pipelines using tools like PySpark or Spark SQL on AWS EMR, offering cost efficiency.
- Databricks might be the most functional for feature engineering and ML training tasks using the same shared data.
- Even business intelligence tasks face competition. Tools like dbt’s semantic layer and Atscale can cache regularly queried metrics while maintaining permissions in sync with the DBMS.
- Shift in governance: The ownership and management of permissions has shifted away from being the sole responsibility of the DBMS.
Challenges Ahead
A significant challenge with modular storage is determining the optimal balance of governance between database management systems (DBMS) or query engines and open storage platforms like Iceberg and other open table formats. Key Issues Posed by George Gilbert- Will modular components such as Iceberg and Starburst complement the incumbent platforms or will customers use them to assemble a full alternative?
- The incumbents are already becoming more modular. They’re not monolithic systems.
- Snowflake is opening up for access by Spark, for example.
- The big question is how long until the components from different vendors work together more easily.
So when when you guys talk about these platforms I think that we’ve already seen the modularity starting to creep in. If you look at just the last three of these Google, Microsoft, Amazon and to a certain extent Databricks and Snowflake coming on board, we’re already seeing that these are not platforms that are a single system, like a single engine, a single storage layer. These are already complex things made of very diverse products. So you’re already seeing engines from the Hadoop space and Microsoft Synapse, right? It’s SPARC, right? SPARC is also part of the Databricks platform. A huge imperative for Snowflake these days is how do we get data to people that want to use Spark with it. And so I think we’ve already started to see these systems decomposing and becoming a collection of projects that all work together rather than one big monolithic system. The question has to do the with all the VC investment that you were alluding to. How how long are we going to wait until all of those components work together really well? And what needs to change.[Listen to Ryan Blue’s opening comments regarding the five modern data platforms].
Assessing the ETR Spending Data on the Big 5 + Oracle
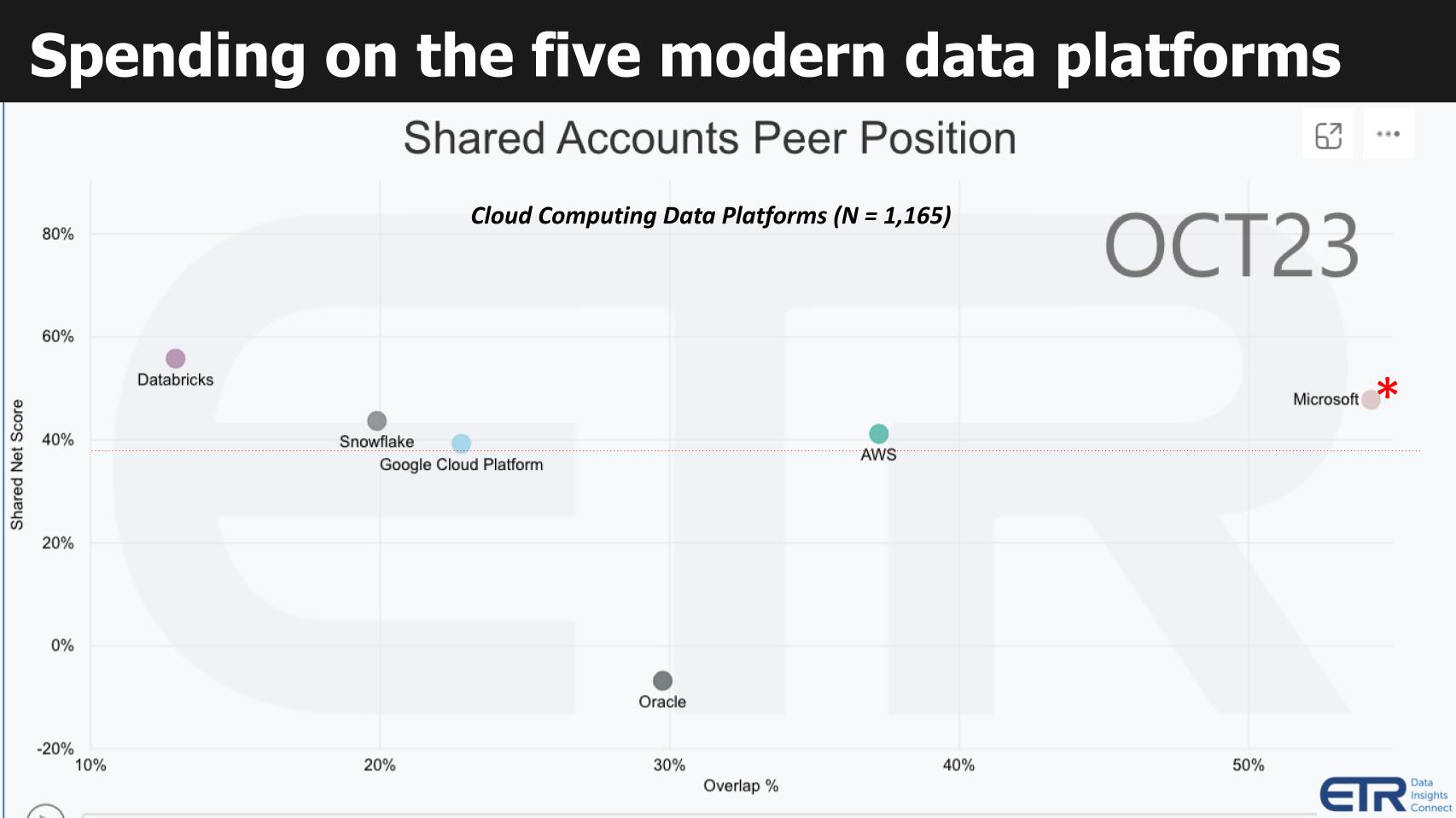
Figure 2: ETR’s Technology Spending Intentions Survey shows spending momentum and market presence for the major data platform vendors. X axis represents account penetration. Y axis represents spending momentum. The graphic above shows spending data within the cloud for the leading data platforms. This specific cut of the data is for database and data warehouse offerings filtered by cloud customers. We include Oracle in deference to the database king, which continues to aggressively invest adding capabilities to its data platforms. It also provides relative context because Oracle has a sizable presence and is mature. The Y axis shows Net Score or spending momentum. It is a measure of the net percent of customers in the survey (N=1,165 cloud customers) spending more on a specific vendor platform. The X axis labeled as Overlap is an indicator of presence in the data set and is determined by the N’s associated with a specific vendor, divided by the total N of the sector. Think of the horizontal axis as a proxy for market presence. The red dotted line at 40% is an indicator of highly elevated spending velocity on a platform. The following key points are notable:
- Virtually all data platforms have seen spending headwinds due to the macro economic environment.
- Microsoft is ubiquitous as shown on the X axis due to its large installed base. There’s probably lots of SQL Server finds its way into the survey (hence the red asterisk).
- Databricks has the highest Net Score but is relatively nascent because its SQL data warehouse is a more recent market entry.
- Over the past several quarters, Snowflake has continued to penetrate the market on the X axis but its Net Score has decelerated dramatically. Based on an analysis of the data, this is due not from customers spending less or churning but rather an increase in the percentage of Snowflake customers shifting from a posture of spending more to one of flat spend.
- AWS’ bespoke set of database services continues to maintain a strong Net Score and AWS’ presence in the data remains impressive.
- Oracle as you can see above has a prominent presence but is essentially living off its installed base.
Emerging Components of the Sixth Data Platform
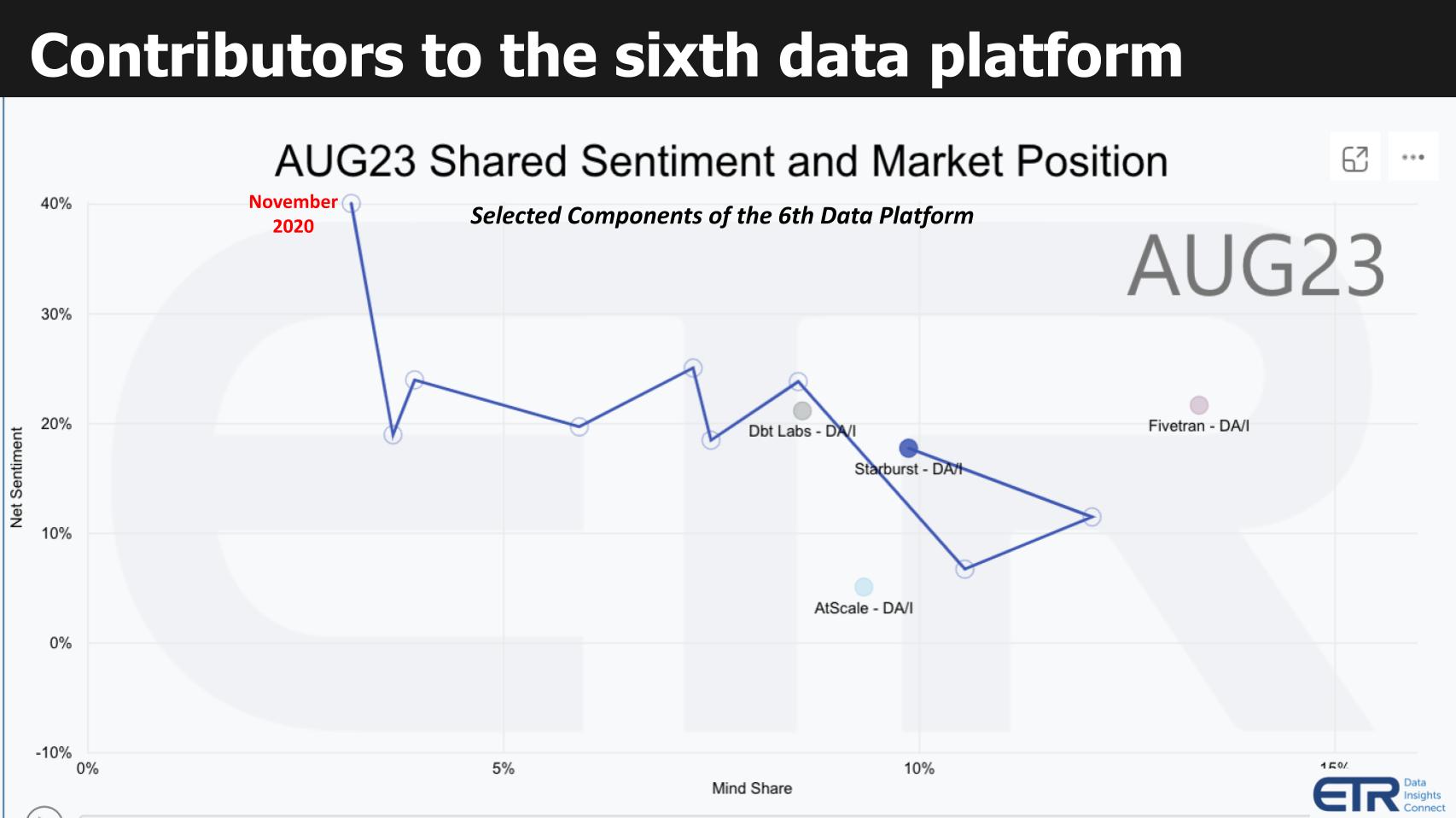
- Net sentiment is a measure of the net percent of customers that intend to engage with the platform. It “nets out,” for example, customers that have no intention of engaging.
- Among components of an emerging 6th data platform, Starburst, dbt, Fivetran, and Atscale show growing mindshare and have respectable or strong sentiment.
- We see companies like these as important contributors and indicators of an emerging sixth platform. Whether they have the funding, vision, execution ethos and staying power to become a sixth platform is unclear at this time.
The Modular Decomposition of Integrated Data Platforms and the Emergence of Multi-Vendor Modules
Key Issues Posed- Are the integrated data platforms modularizing or are a bunch of multi-vendor components emerging – or both?
- What use cases are drawing these multi-vendor components into the market?
- Snowflake started out as an integrated system but added progressively deeper Iceberg support – what does this imply?
- Do markets like this start with integrated products and then modularize and what does that mean for the future of data platforms?
The integrated vendors supported open storage because they wanted access to more data But once customers can use open formats, their data is no longer trapped in vendor silos. If you take both Databricks and Snowflake, they want access to all of the data out there. And so they are very much incentivized to add support for projects like Iceberg. Databricks and Snowflake have recently announced support for Iceberg, and that’s just from the monolithic vendor angle, right? I don’t think anyone would have expected Snowflake to add full support for a project like Iceberg two years ago. That was pretty surprising. And then if you look at the other vendors, they’re able to compete in this space because they’re taking all of these projects that they don’t own and they’re packaging them up, as, you know, out of the box data architecture. One of the critical pieces of this is that we’re sharing storage across these projects, and that has for the data warehouse vendors like Snowflake, the advantage that they can get access to all the data that’s not stored in Snowflake. But I think a more important lens is from the buyer or the user’s perspective where no one wants siloed data anymore and they want a essentially what what Microsoft is talking about with Fabric. They want to be able to access the same datasets through any different engine or means, whether that’s a Python program on some developer’s laptop or a data warehouse at the other end of the spectrum like Snowflake. So it’s very important that all of those things can share data. Of course, that’s that’s where I’m coming from. That’s what I’m most familiar with. You know, if you go above the the layer of those engines, I’m less familiar, but we even see that that consolidation with integrations like Fivetran writing directly to Iceberg tables.[Listen to Ryan Blue’s comments on the shift to modularity, adoption of Iceberg, data sharing & market surprises].
Five Key Questions Related to the Emergence of a Sixth Data Platform
In this next section we review critical questions that we feel are central to understanding the emergence of a sixth data platform. We’ll combine a summarization of comments with verbatim statements and provide video links to specific sections of the conversation.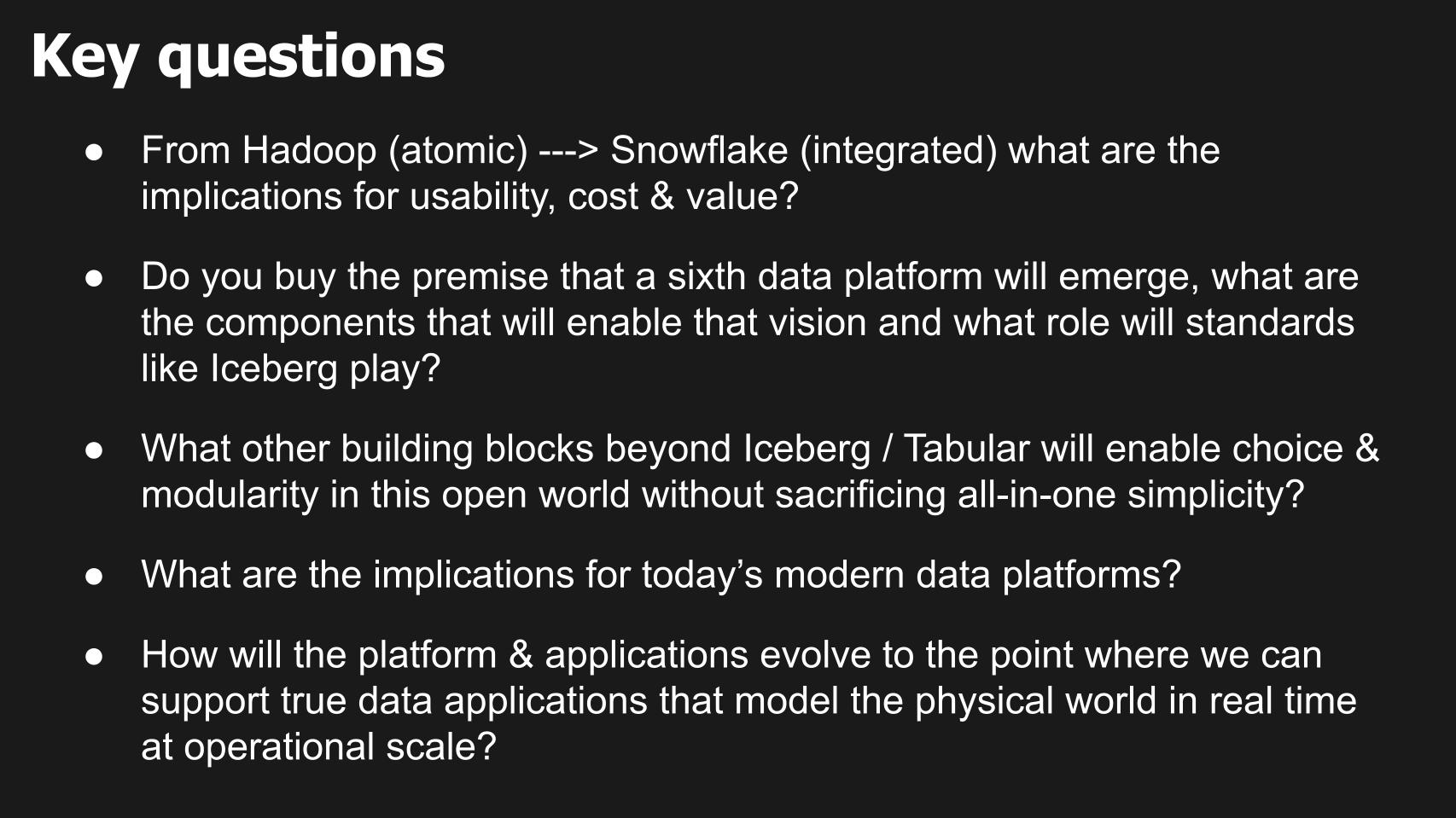
Figure 4: Key questions Ryan Blue of Tabular addresses about multi-vendor data platforms with open storage. In the ever-evolving world of data platforms, we saw the journey from the atomic and fragmented Hadoop ecosystem to the highly integrated systems of today, exemplified by Snowflake. We see a future of more pivotal shifts. Recent trends raise pivotal questions about usability, cost, and value in the data landscape.
- From Hadoop to Snowflake: Hadoop’s complex architecture was service-heavy and required specialized expertise, often from external consultants or in-house experts in proverbial “lab coats.” Despite its promise, Hadoop struggled to gain substantial traction due to multiple factors:
- Complexity.
- Disruption from Apache Spark.
- The cloud’s increasing dominance.
- The Snowflake Proposition: With its integrated approach, Snowflake presents a unique value proposition. However, insights gleaned from the recent Snowflake Summit suggest some tension:
- Ecosystem partners and customers increasingly indicate that organizations are conducting data engineering work outside of Snowflake, primarily due to cost concerns.
- Snowflake counters this by emphasizing the full value attained when such operations are performed inside Snowpark, championing the integrated total cost of ownership (TCO) benefits of Snowflake’s approach.
- The Current Landscape:
- ETR data points to a recent deceleration in Snowflake’s momentum, especially concerning the percentage of customers spending more and new customer adds.
- Yet, Snowflake’s integrated value proposition remains a strong selling point.
- Storage shared by multiple products is the biggest change in DBMS architecture and business model in more than a decade.
- Even though storage and compute were architecturally separate, they were de facto integrated by the vendors.
- Once a vendor owned your data, they owned all your future workloads.
- The new modularity inherits the spirit of the Hadoop world where you assumed batch, streaming, and ad-hoc engines all shared the same data.
- That shared data storage is the assumption behind Iceberg.
I do believe in the modular world. I think that the biggest change in databases in the last ten years easily, if not longer, is the ability to share storage underneath databases. And that really came from the Hadoop world because those of us, we weren’t wearing lab coats, but those of us over there, we sort of had as an assumption that many different engines, whether they’re streaming or batch or ad hoc, they all needed to use the same data sets. So that was an assumption when we built Iceberg and other similar formats. And that’s what really drove this change to be able to share data. Now in terms of cost and usability, that is a huge disruption to the business model of basically every established database vendor who has lived on the Oracle model. And it’s not really even the Oracle model. It’s just that storage and compute have always been so closely tied together that you couldn’t separate them. And so by winning workloads, winning datasets, you also were winning future compute whether or not you were the best option for it, best option meaning what people knew how to use best in terms of performance and cost and things like that. And so the the shift to sharing data means we can essentially move workloads without forklifting data, without needing to worry about how do we keep it in sync across these products, how do we secure it. All of those problems have been inhibitors to moving data to the correct engine that is the most efficient or the most cost-effective, etc. So I think that this shift to open storage and independent storage in particular, is going to drive a lot of that value and cost efficiency.[Watch and listen to Ryan Blue’s commentary on sharing underlying storage].
Key Issue: What’s the Ideal Governance Approach in a Shared Storage Model
George:- Once you have shared storage separate from compute, something has to manage transactional consistency, permissions, and broader governance policies.
- Data lakes have a massive gap.
- In order to share data between any compute engine, storage now has to own permissions.
- Iceberg is clearly a foundational component of this sixth data platform.
- But the rest still seems a little fuzzy.
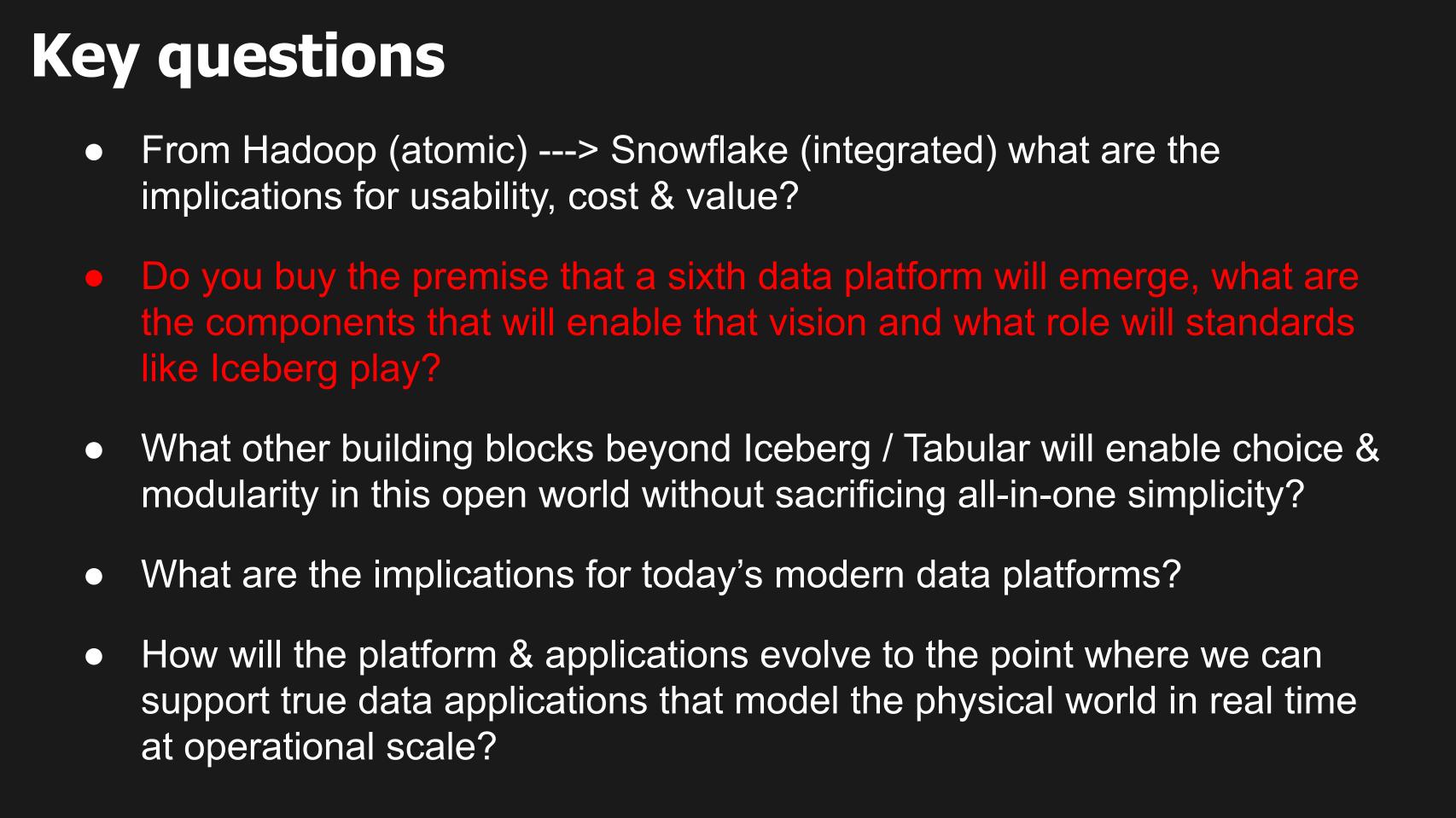
- The sixth data platform is probably not going to be a distinct product.
- All incumbent vendors are probably going to standardize on certain components
- If Snowflake and Databricks can agree on Iceberg, that’ll likely be the standard for everyone
- We still have to standardize things like views, encryption, catalog interaction, etc.
- The sixth data platform is going to be all players sharing data within the context of the same architecture.
Yeah, I think that the sixth data platform is as good a name as you can come up with, right? I don’t think that we know what it’s going to be called quite yet. I don’t know that I would consider it distinct because I think what’s going to happen is all five of those players that you were talking about, plus Oracle plus IBM and others, are going to standardize on certain components within this architecture. or Certainly shared storage is going to be one. And I believe that Iceberg is probably that shared storage format because it seems to have the broadest adoption. You know, if Databricks and Snowflake can agree on something, then that’s probably the de facto standard. We are still going to see what we can all agree on as we build more and more of those standards in the Iceberg community. We’re working on shared things like views, standardized encryption, you know, ways of interacting with a catalog, no matter who owns or runs that catalog. And I think those components are going to be really critical in the sixth data platform because it’s going to be an amalgam of all of those players sharing data and sharing or being part of the exact same architecture.[Listen and watch Ryan Blue discuss his view that virtually all major data platforms will adopt open, modular standards]. Dave:
- As the data platform evolves to support more real-time or operational applications, we can’t count out Oracle.
- But applications that look more like Uber are still some years out.
- We still need to see relational knowledge graphs and transactional relational document databases mature.
How to Maintain Data Integrity. Which Layer of the Stack is Responsible?
George:- What goes in the storage manager?
- How do you manage transactional integrity?
- How far up the stack do you go?
- Iceberg and Delta already have transaction protocols.
- But table updates currently have an update latency challenge that favors throughput.
- We still have to figure out how to merge streaming data with historical data.
Yeah. So I think you you brought up a couple of different areas, and I’ll address those somewhat separately. So first of all, in terms of transactions, that’s one of the things that the open data formats, and I’m including just Delta and Iceberg in that, what they do is essentially have a transaction protocol that allows you to safely modify data without knowing about any of the other people trying to modify or read data there. The two formats do that and are open source. So that I think is a solved problem. Now, the the issue that you then have is they do that by writing everything to an object store and cutting off a version, which is inherently a batch process. And that’s where you start having this mismatch between modern streaming, which is a micro-batch streaming operation and efficiency. Because you need to, you know, at each point in time commit to the table, every single commit incurs more work or something like that. So in order to get towards real-time, you’re simply doing more work and you’re also adding more complexity to that process. So I think that essentially you’re seeing cost rise at least linearly, if not exponentially, as you get closer and closer to real time. I think that the the basic economics of that makes it infeasible for in the long term to really make that real-time something that you’re going to use 100% of the time. And this is the age-old tradeoff between latency and throughput. If you want lower latency, you have lower throughputt. If you’re willing to take higher latency, you have higher throughput and, thus better efficiency. So I think that where we need that streaming and the sort of constantly fed data applications, those are going to get easier to build, certainly. But I think that after those challenges is probably where you’re going to go and store data, make it durable for this sixth data platform. And it’ll be interesting to see the interplay between those real-time sort of streaming applications and how we sort of merge that data with data from the lake or warehouse.[Watch a clip of Ryan Blue addressing some of the challenges, tradeoffs and costs associated with handling different workload types, data formats and getting to a real time model].
What are the Building Blocks Beyond Tabular and Iceberg to Deliver Comparable Value to an Integrated System…A Netflix Example
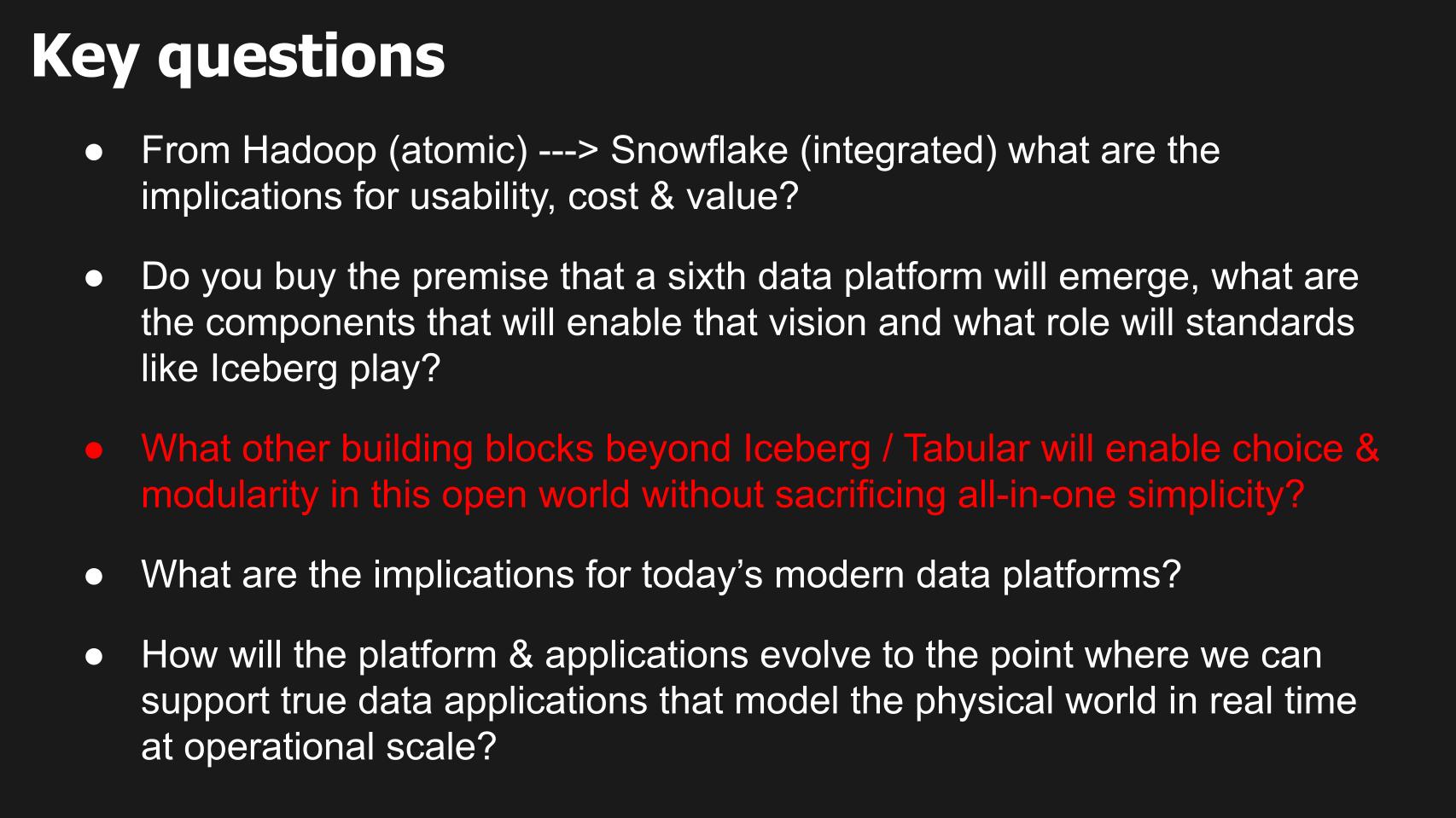
- We want to explore the belief that data applications that orchestrate the real world like Uber are going to be mainstream.
- What are the missing building blocks, especially with respect to today’s modern data stack?
- It sounds like if you want a real-time system of truth, you’re going to use an operational database?
- And you’re going to use a separate historical system of truth?
- The way we are tearing down the data silos between platforms is essentially to trade-off some of the machinery that would support real-time applications – at least in the short-term.
- We won’t merge those worlds any time soon. But we can make it easier to use real-time and historical systems together.
- We did that at Netflix. The developer could get data that was milliseconds old as well as two years old nearly seamlessly.
How to Hide the Differences Between Real-time and Historical Domains at the Developer Level
George:- How were you able to hide the difference between real-time and historical domains from the developer at Netflix?
- At Netflix the application back-end was responsible for receiving all the logs almost in real-time and managing the hand-off between Iceberg for historical data and in-memory for real-time data.
Through building a data app that was a ton of work and understood both of those data storage domains. So the app or the back end, at least, was responsible for receiving all of the logs in a almost real time and for storing them and managing that handoff between in-memory and iceberg.George:
- Help us understand exactly what governance data, including permissions and technical metadata, goes into storage.
- What metadata stays outside storage?
- We distinguish between technical metadata to read and write Iceberg data and higher-level operational metadata that’s separate.
In terms of Iceberg, we do make a distinction between technical metadata required to read and write the format properly and higher level metadata. And that higher level metadata we think of as business operations info, even things like access controls and our back policy and all of that. That’s a higher level that we don’t include. And even the Iceberg open source project, the technical metadata is quite enough to manage for an open source project.
Implications for Today’s Modern Data Platforms
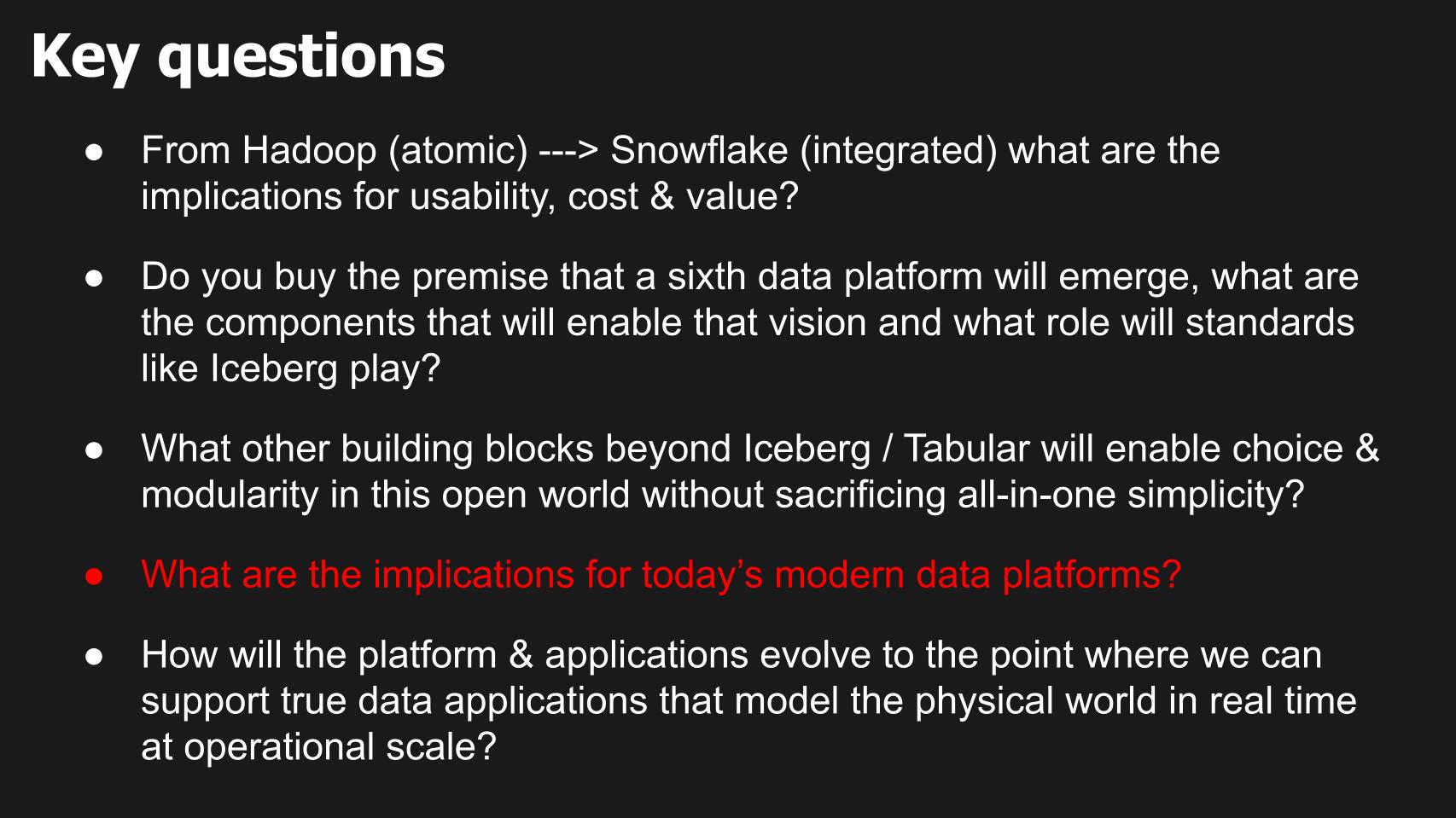
- The business model of data management changes. Vendors have to compete for every workload.
- Is metadata management (an operational catalog) the new gateway or chokepoint for data management?
- Access controls and certain governance operations need to move into the storage layer catalog that is standardized.
- Beyond that, it’s still unsettled.
- But we need to solve the problem of customers copying data between vendor platforms today.
I think that it is absolutely critical to have a plan around that metadata. We don’t really know how far we’re going to go down that road. So I think that today there’s a very good argument that access controls and certain governance operations need to move into a catalog and storage layer that is uniform across all of those different methods of access and compute providers. What else goes into that, I think is is something that we’re we’re going to see. I think that that is by far the biggest blocker that I see across customers that I talked to. You know, everyone has already Databricks and Snowflake and possibly some AWS or Microsoft services in their architecture and they’re wondering how do I stop copying data? How do I stop syncing policy between these systems? I know that we need to solve that problem today. But the higher level stuff, because usually, you know, like we work with a financial regulator that has their own method of tracking policy and translates that into the role-based access controls underneath.
You know, how you manage that policy may be organization specific, it might be something that that evolves over time. I think it’s you know, we’re we’re just at the start of this market where people are starting to think about data holistically across their entire enterprise, you know, including the transactional systems, how that data flows into the analytics systems, how we re secure it and have the same set of users and roles and potentially access controls that follow that data around.
This is a really big problem and I wish I had a crystal ball, but I just know that the next step is to to centralize and have fewer permission systems and, you know, just one that maybe covers 95% of your analytic data is going to be a major step forward.[Listen and watch Ryan Blue discuss the future of data management and the unknowns on the horizon].
The Power of Inertia: How will Data Platforms Evolve Given Today’s Customer Preferences?
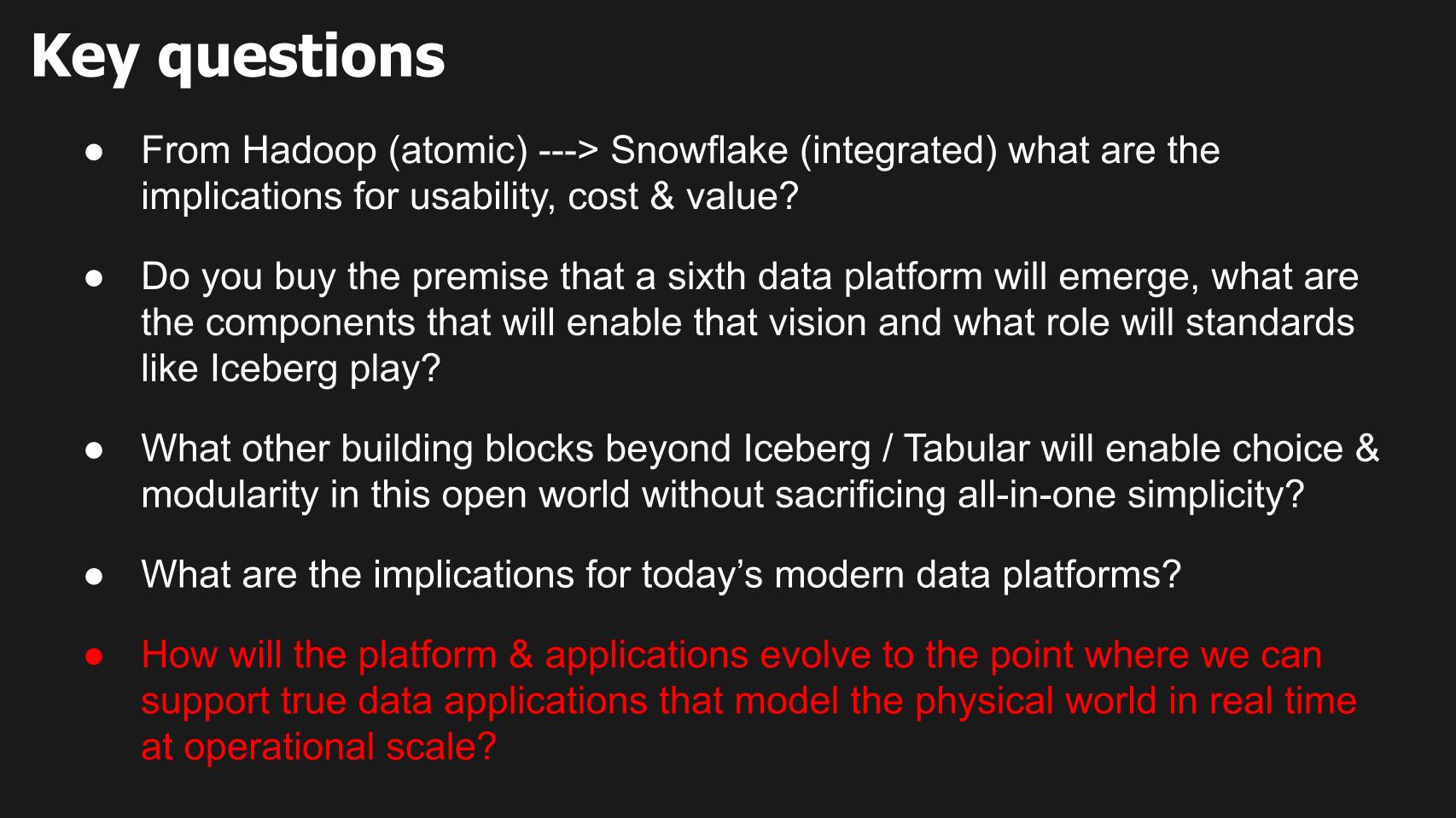
- The world is moving to a data-centric world from a data platform-centric world.
I think right now companies have a different problem. It’s not like they’re they’re looking at this and saying, who? I want to move this workload. Or maybe they are, but it depends on where they’re coming from. If you already have Spark and you need a much better data warehouse option, then you might be adding snowflake. You might also be coming from Snowflake and going towards ML in Spark. You know, those, those sorts of things. I can’t really summarize that. I would say that the biggest thing that we see in large organizations is that you have these pockets of people that, you know, this group really likes Spark. This group is perfectly happy running on Redshift. Someone else needed the performance of Snowflake and the CIO level is looking at this as what is our data story? How do we fix this problem of needing to sync data into five different places? I was talking to someone at our company that used to work in ad tech just this morning and he said that they had, you know, five or six different copies of the exact same data set where different people would go to the same vendor, buy the dataset, massage it slightly differently to meet their own internal model and then use it. And it’s it’s those sorts of problems that it’s like, let’s just store it once and like, let’s store all of this data once and make it universally accessible. We don’t have to worry about copies, we don’t have to worry about silos, we don’t have to worry about copying governance policy. And is it leaking? Because, you know, Spark has no access controls. While I’ve locked everything down in, say, Snowflake or Starburst. It’s just a mess and so the first thing that people are doing is trying to get a handle on it and say, we know we need to consolidate this. We know we need to share data across all of these things. And thankfully, we can these days. Ten, ten years ago, the choice was we can share data, but we have to use Hadoop and it’s unsafe and unreliable and very hard to use.Or we can continue having both Redshift and Snowflake and Netezza in our our architecture, So we’re we’re just now moving to where it’s possible and we’re we’re discovering a lot along the way here.[Ryan Blue discusses the key issues customers face, inertia and the challenges of syncing data that lives in many places].
Integrated Simplicity Vs. Open Modularity: Complement of Competition
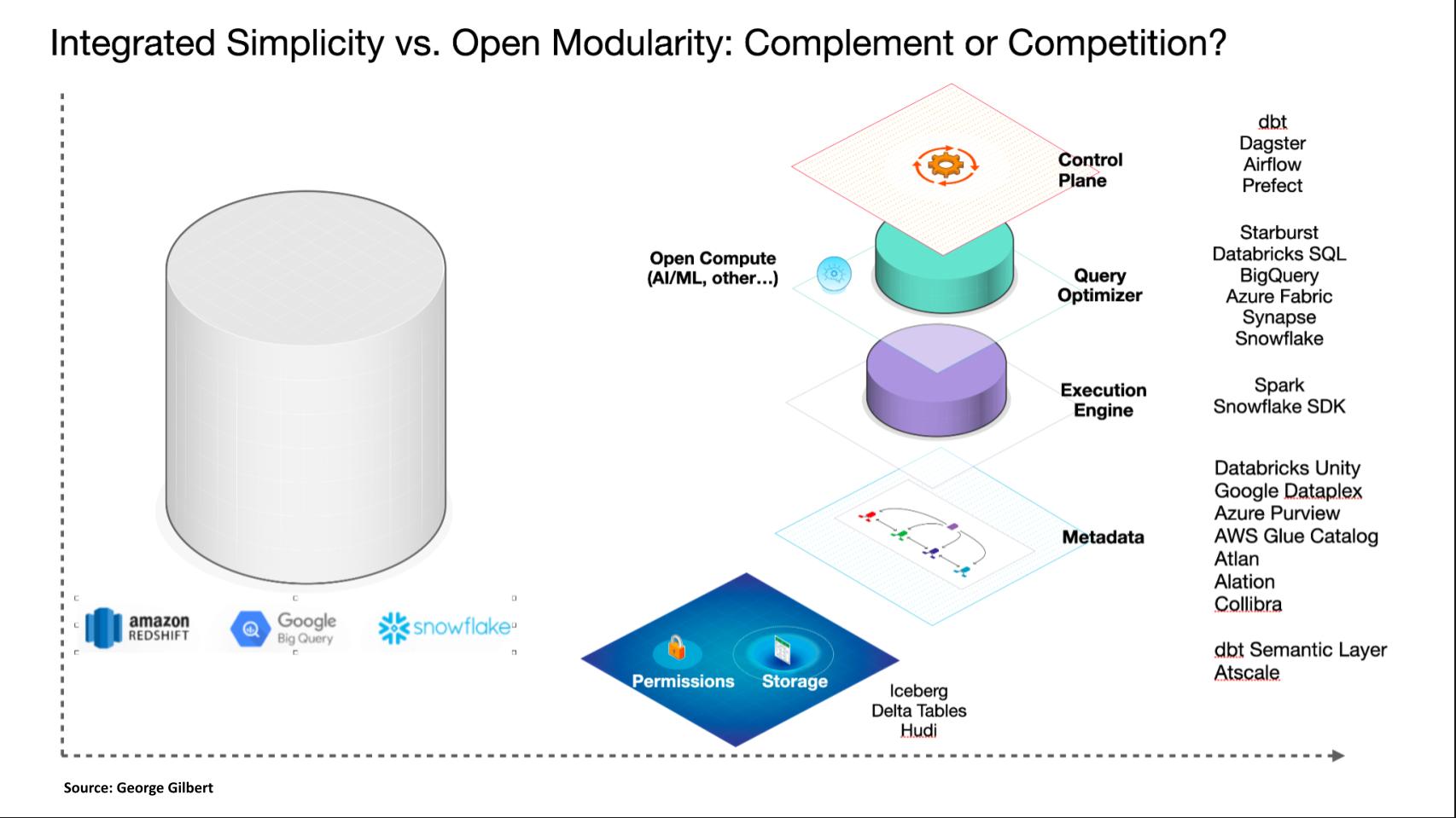
Final Thoughts by Ryan Blue
That’s the big question. How are we getting there? Do we have the components right? What is it all going to look like when we get there? It has big implications for for the products customers buy and for the business model of the vendor selling to them. Ryan:- We need both modularity and simplicity.
- Database services from the query layer are moving to the storage layer.
- Simplicity of access for OAuth is a key initiative.
- Lessons from Hadoop’s failings.
So just the high level modularity versus simplicity, I think we absolutely need both, right? The modular it is is clearly being demanded. The simplicity always follows afterwards. You know, databases, power applications. So there’s always been a gap in code and who controls what. And and these things, we’re just adding layers. We pretty much know the boundaries between a database and an application on top of it and making that a smooth process. I think that we’re doing that again, separating that storage and compute layer. But we absolutely need both. And this is where some of the newer things that we’ve been doing in the Apache Iceberg community come into play. Standardizing how we talk with catalogs, making it possible to actually secure that layer and say, ‘hey, this is how you pass identity across that boundary.’ We’re also moving database services from the query layer for maintenance into the storage layer. That’s another thing that’s moving…that modularity needs to be followed by the simplicity, for instance things that use OAuth. We’re pioneering a way to use OAuth to connect the query engine to our storage layer so that you can just click through and have an administrator say, ‘Yes, I want to give access to this data warehouse, to Starburst’ or something like that. That ease of use, I think is is really the only thing that is going to make modularity happen because, I mean, again, the big failure of the Hadoop ecosystem was that simplicity and that usability we have. We’ve only been able to see the benefits of that by layering on and maturing those products to add the simplicity. And so that’s absolutely a part of where we’re going.[Listen to Ryan Blue summarize his final thoughts on the conversation].
Keep in Touch
Many thanks to Alex Myerson and Ken Shifman on production, podcasts and media workflows for Breaking Analysis. Special thanks to Kristen Martin and Cheryl Knight who help us keep our community informed and get the word out. And to Rob Hof, our EiC at SiliconANGLE. Remember we publish each week on Wikibon and SiliconANGLE. These episodes are all available as podcasts wherever you listen. Email david.vellante@siliconangle.com | DM @dvellante on Twitter | Comment on our LinkedIn posts. Also, check out this ETR Tutorial we created, which explains the spending methodology in more detail. Watch the full video analysis:Image: George Gilbert
Note: ETR is a separate company from Wikibon and SiliconANGLE. If you would like to cite or republish any of the company’s data, or inquire about its services, please contact ETR at legal@etr.ai.
All statements made regarding companies or securities are strictly beliefs, points of view and opinions held by SiliconANGLE Media, Enterprise Technology Research, other guests on theCUBE and guest writers. Such statements are not recommendations by these individuals to buy, sell or hold any security. The content presented does not constitute investment advice and should not be used as the basis for any investment decision. You and only you are responsible for your investment decisions.
Disclosure: Many of the companies cited in Breaking Analysis are sponsors of theCUBE and/or clients of Wikibon. None of these firms or other companies have any editorial control over or advanced viewing of what’s published in Breaking Analysis.