Contributing Wikibon Analysts
Ralph Finos
David Floyer
George Gilbert
Brian Gracely
Peter Burris
Premise
Big data and public cloud share a natural affinity, but are not a perfect match. Doers should explore public cloud options for their big data workloads because public cloud offers a simple way to scale up and down workloads running mostly using open source software. However, moving large data sets over distance remains an intractable cost. The growth of big data workloads in the public cloud will closely follow the evolution of technology for staging, pipelining, testing, and administering big data.
The market for big data in the public cloud in 2015 (Figure 1) was $1.1B (5% of all big data revenue) and will grow to $21.8B by 2026 (24% of all big data revenue). In terms of overall public cloud spending, big data represented 1.4% of public cloud revenue in 2015, but will grow to 4.4% by 2026 (see Figures 2 and 3). The characteristics of public cloud are an especially good fit for big data workloads and this will be accelerated as the software ecosystem matures and data and analytics of all types pivots more to the cloud.
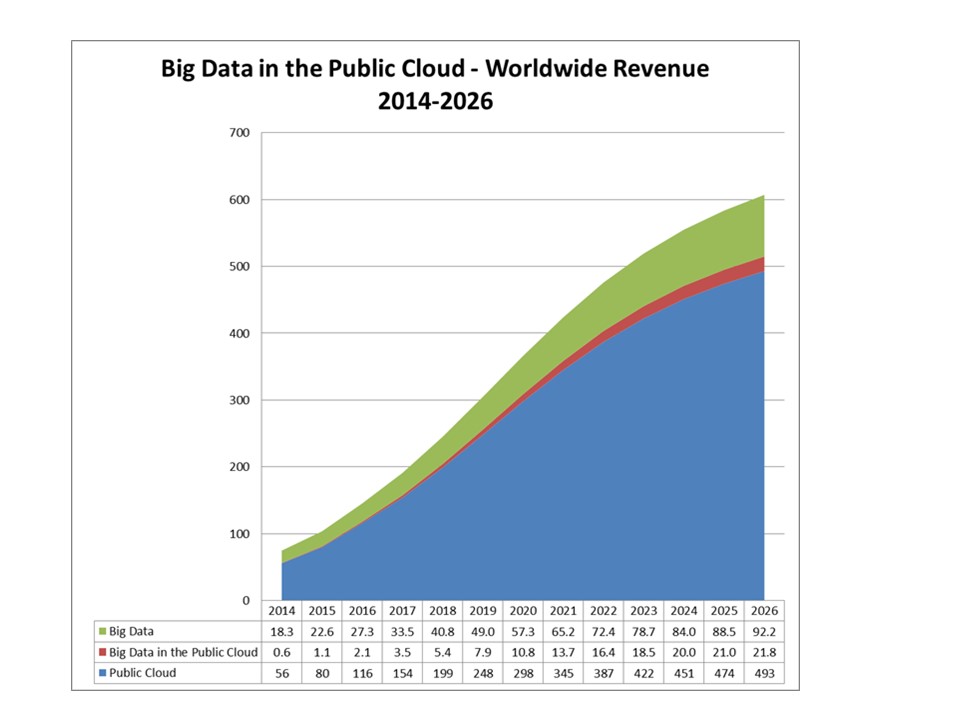
Source: Wikibon Big Data in the Public Cloud Forecast, Wikibon May 2016 Report
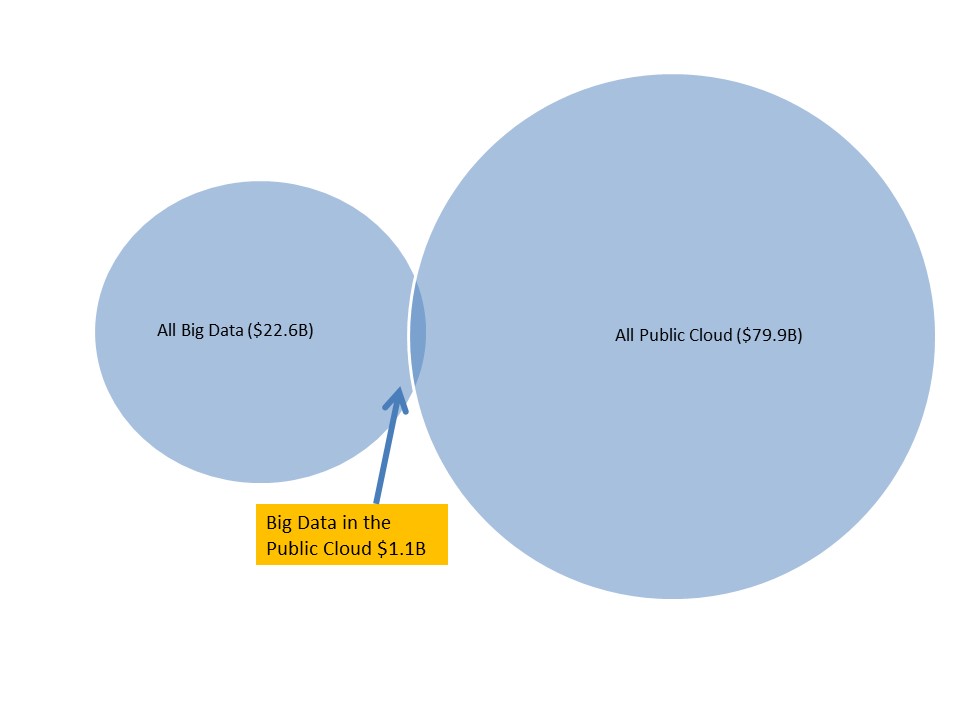
Source: Wikibon Big Data in the Public Cloud Forecast, Wikibon May 2016 Report
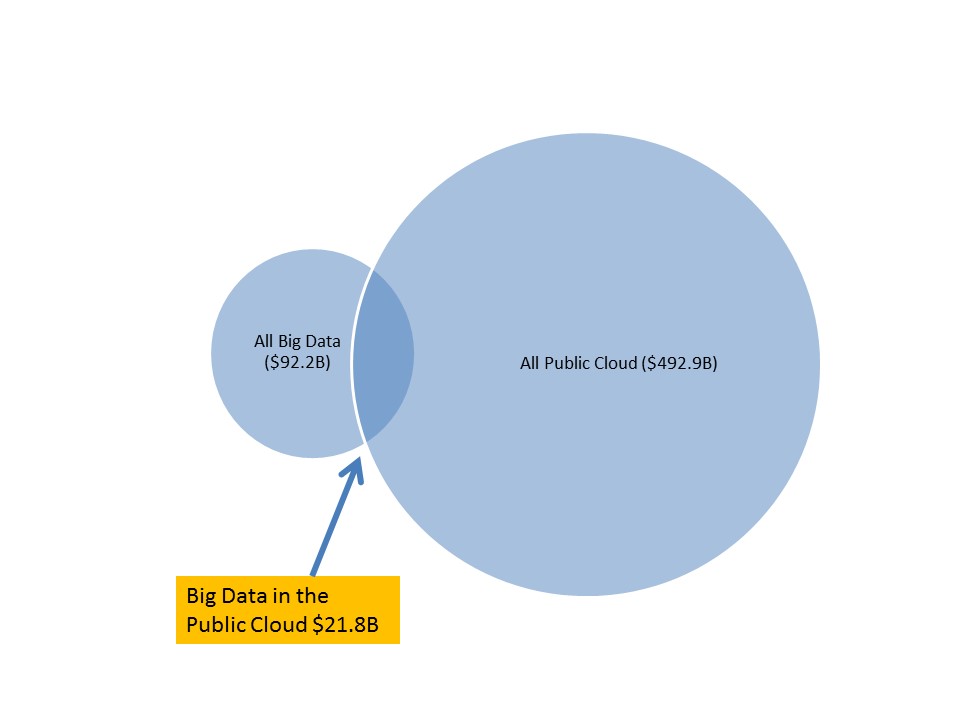
Source: Wikibon Big Data in the Public Cloud Forecast, Wikibon May 2016
Generally, versions of the most popular big data software tools are available in the public cloud; the tools will only gain greater function as the market matures. Many of the top 30-40 big data software providers today have offerings available on AWS and/or Azure. In our interviews with these vendors many reported public cloud-based revenue in the 5-10% range today. Hortonworks, Cloudera, and MapR collectively indicated that >10% of their 2015 revenue came from the public cloud.
Big data workloads in the public cloud today are primarily deployed in AWS and Microsoft Azure. For example, a forthcoming Wikibon review of ~650 case studies profiled on AWS’s website (https://aws.amazon.com/solutions/case-studies/all/) suggests that as many as 10% of them were doing some form of big data from adtech to data center log analytics to smart grid to health care apps. Airbnb, Lyft, Yelp, Splunk, et alia are among those profiled by AWS doing big data workloads. Salesforce just recently joined these vendors in deploying at least one of its workloads on AWS. Microsoft Azure has similar examples from their customer case studies. The breadth and depth of successful big data workloads in the cloud is extensive and it will continue to expand.
Big data’s future in the public cloud is strong. In our interviews, big data software vendors often indicated their public-cloud software revenues had doubled in 2015 with similar expectations for 2016. Big data apps will garner a larger share of overall big data spend (including dollars currently targeting on-premises and hardware, software, and services) as well as tripling its share of overall public cloud spending by 2026 with growth coming largely from marketing, adtech, social and business networks, mobile, and similar apps. Even with this impressive growth, big data’s share of public cloud is small and will remain so throughout the next decade. This is largely due to the fact that there are no full-scale SaaS apps in big data today that compare to the traction and market power of Salesforce, Oracle, and SAP as well as the many collaborative applications that are a good fit for public cloud deployment. However, our big data in the public cloud growth rate assumes there will be full scale big data SaaS applications in the public cloud in the out years of the forecast.
Same Old Drivers for Public Cloud Exploitation…
The market drivers for big data in the public cloud are the same as those for any workload or application – cost transparency, scalability, rapid deployment, ease-of use, and no visible IT admin to contend with acquire resources. Public cloud is especially attractive to companies featuring a value proposition that is tied directly to cloud-resident data sets, such as adtech, social and business networks, ecommerce, and other customer/outward-facing applications. The growth of big data in the public cloud is also benefiting from the acceptance of analytics-as-service providers serving small and medium businesses, start-ups, and increasingly large enterprises.
For enterprises large and small, discovery, developer sandboxes, and big data proofs-of-concept are less risky and more transparent in the public cloud than on-premises. Large enterprises can find the public cloud to be a useful adjunct for off-loading low value infrastructure workloads or data sets. All these factors work to the advantage of big data deployment in the public cloud – especially in terms of scalability, low cost storage of the former, and ease of use and admin (given the difficulty of deploying big data tools and the dearth of available data science skills that plagues this market). Lastly, purchasing services is less risky, cost-wise, than deploying a complex set of big data tools especially in the early stages of experimentation and proofs of concept.
The attractiveness of deploying big data analytics in the public cloud is amplified by the fact that big data workloads tend to be highly departmental in nature. In our Fall 2015 Big Data survey http://wikibon.com/wikibon-big-data-analytics-survey-fall-2015/ we found that business analysts were more interested in public cloud than practitioners in other big data roles. Marketing departments represent the most frequent public cloud use cases today (e.g., adtech, customer segmentation, social media analysis, recommendation engines). Operations departments utilizing log files and sensor data in process manufacturing industries are other examples of the sorts of departmental practitioners who find the public cloud more appealing than on-premises solutions.
…But the Barriers are More Unique
The hurdles to big data in the public cloud are more daunting compared to other workloads – at least in the near term. Infrastructure complexity for well-understood workloads largely is masked for buyers of public cloud services; for less well-understood workloads, like rapidly evolving big data workloads, firms still need to master the management of public cloud resources, big data software toolset configurations, job and workload management, and the financial risks of large scale resource utilization. Larger enterprises may opt to buy hosted cloud services from providers like Teradata and SAS Institute who provide better-understood BI and data warehouse applications.. Smaller enterprises may opt for hosted solutions as well if they lack the skills to use big data effectively.
Customers choosing integrated big data analytics and management software from public cloud vendors can avoid the challenge of administering the mix and match collection of ill-fitting Hadoop components. Cloud-native products, by contrast, are designed, built, tested, and operated to work as one integrated set of services. Over the next few years, users will have to trade-off the benefits of integrated tools with the potential costs of lock-in, but today we believe the integration benefits, given experience challenges, outweigh lock-in costs, given the prevalent use of open source software.
The second significant barrier to big data in the public cloud won’t go away with experience: The cost to move huge volumes of data go up at least linearly, because of physics. As data volumes grow, pressure to rationalize data movement will grow, as well. Over the next few years, greater understanding of edge-based architectures will offer important options as edge-based products, design methods, and operational practices emerge and evolve. Our forecast, however, presumes that this edge-based options will mature quite rapidly, ameliorating the pressure to move all data into central cloud-based locations.
The third barrier may or may not diminish with experience: Governments want data on their citizens and businesses to stay within national borders. While buying public cloud services for big data workloads in multiple countries will be an option, big data “wants” to aggregate data in ways that doesn’t reflect geographic location. As privacy laws become stronger — and we believe they will — constraints based on national location will increasingly affect big data architectures
Big Data Application Patterns in the Public Cloud
Wikibon’s research indicates that big data workloads will unfold in three waves over the next 10 years: (1) Data Lakes, (2) Intelligent Systems of Engagement, and (3) Self-Tuning Systems of Intelligence) (http://wikibon.com/forecasting-big-data-application-patterns/) The affinity of these workloads for the public cloud vary in the following ways (see Figure 4):
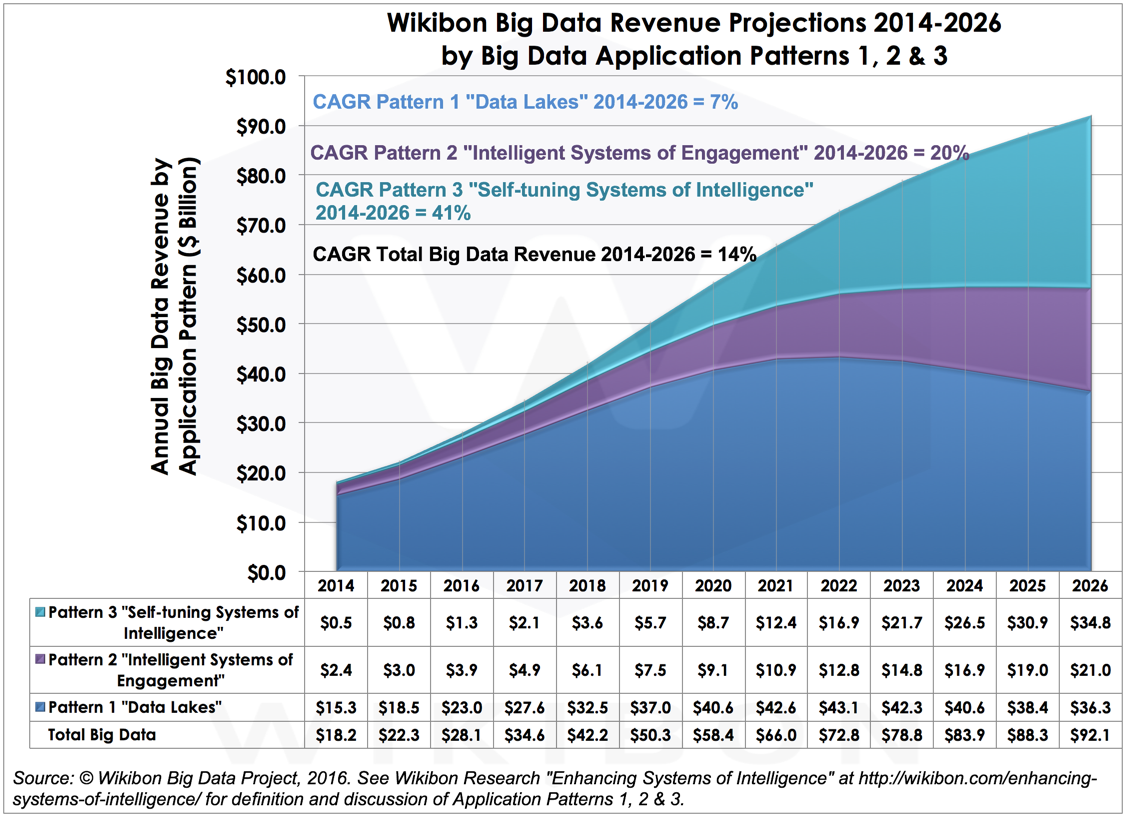
- 2016-2019: Refining Data Lakes. Hadoop has been the main engine of the development of data lakes for data discovery and exploration and it will continue to be driven hard in this role throughout the remainder of this decade. However, the Hadoop ecosystem is comprised of several dozen products that are not at all well integrated. While this problem of an easy to use manageable ecosystem is being solved, the public cloud offers a coherent deployment environment that — while not as rich — is a foundation for solving big data business intelligence and analytic workloads today, especially for data scientists and business analysts seeking to avoid the headaches of complex infrastructure administration. However, because of physical and regulatory constraints, not all data lakes will end up in the public cloud.
- 2020-2022: Maturing Intelligent Systems of Engagement. The new front door for all consumer-facing vendors and services is digital. In addition, digital advertising is growing rapidly which means that the locus of customer transactions and experiences will gravitate increasingly to the public cloud from the start. With engagement data already resident in the public cloud, a sizeable percentage of social, locational, and transactional analytics workloads will best be hosted there, thereby avoiding incurring data movement costs.
In the 2020 period, the capability of Spark to facilitate streaming, machine learning, geospatial, and graph processing will enable big data apps involving customer engagement and relationships to become more real time and more effective than the engagement technologies we have today. Streaming technologies will also impact ETL investments, forcing legacy batch-oriented ETL workloads to be modernized.
- 2022 and Beyond: Self-Tuning Systems of Intelligence. Applications in this period will include predictive analytics, disaster avoidance, risk modeling, and proactive repair of complex systems. The Internet of Things (IoT) — especially sensor-based applications and event processing — will enable self-tuning systems to react to and anticipate system conditions and adjust behavior to optimize performance. Given the real-time characteristics of the (IoT) use cases (i.e. smart utility grids, smart cities, self-tuning industrial equipment, self-driving vehicles), data movement costs will be especially impactful on designs; a significant amount of the apps and data will be primarily at the edge where data will have to be local to the system to be of use. As such, a high percentage of the data processing and management necessary to achieve the benefits will also be local; public cloud will be used for reporting relatively smaller amounts of summary data and exceptional data across multiple edge locations. The principle of moving the computing to the data will minimize costs, and additional analysis will usually be pushed out to the edge, rather that moving the data to the compute.
How Does the Big Data Application Pattern Mix in the Public Cloud Evolve from 2015-2026?
In 2015 Data lakes (43%) comprised a significant amount of big data-related spending in the public cloud in 2015 (Figure 5). Development work (29%) (also including proofs of concept and exploratory discovery work) and Systems of Engagement (28%), i.e. cloud advertising, social networks, and e-commerce are also significant big data workloads in the public cloud. In 2015 Self-tuning Systems of Intelligence was <1%.
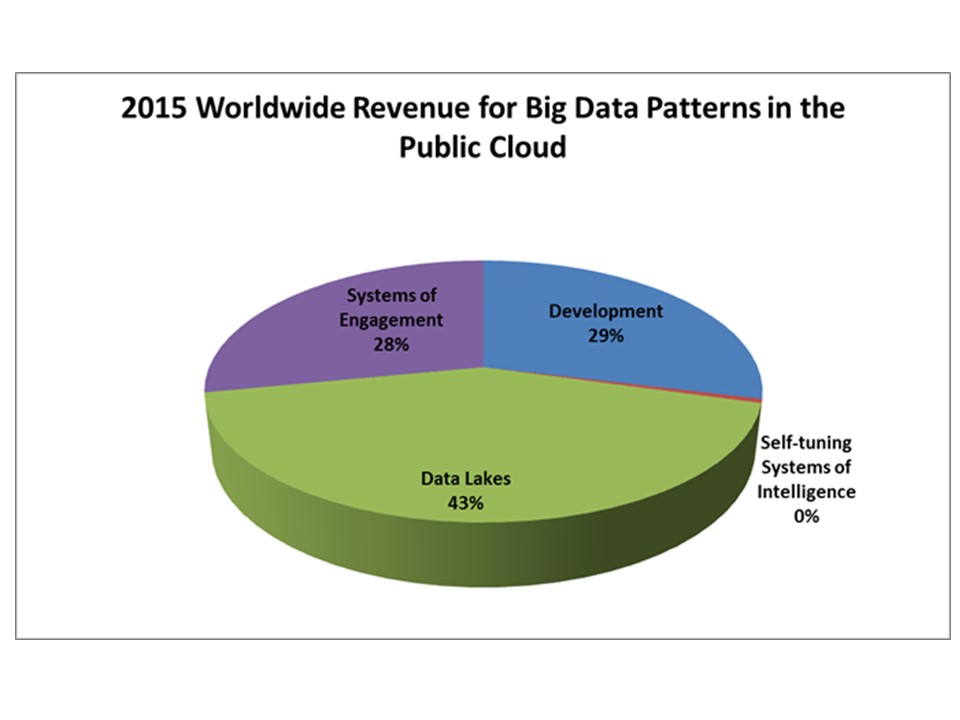
Source: Wikibon Big Data in the Public Cloud Forecast, Wikibon May 2016 Report
In 2026 (Figure 6), Systems of Engagement (45%) will account for the most big data revenue in the public cloud since engagement-related data – advertising, social networks, transactions, etc. – increasingly will reside there. Data lakes will shrink in importance to 25% of spending for big data in the public cloud. Development work will still be an important use case (20%). Self-tuning Systems of Intelligence will still have the least share (10%) of big data spending in the public cloud, with most of that spending involving big data being used for cloud analytics of KPIs of user experience, industrial and smart grid performance and planning, risk management, and others.
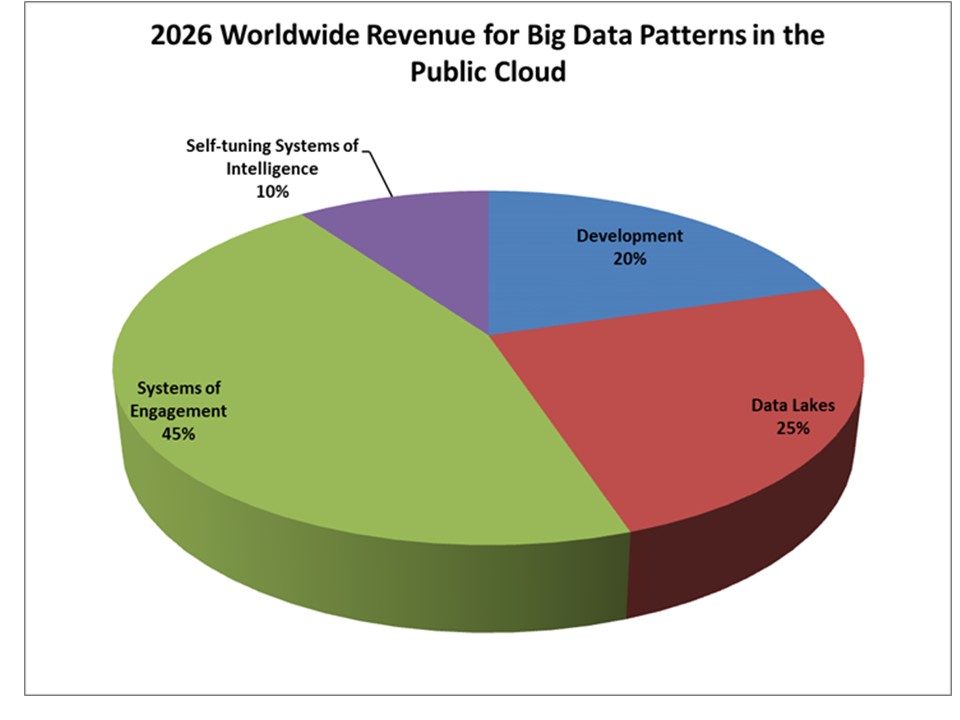
Source: Wikibon Big Data in the Public Cloud Forecast, Wikibon May 2016
Action Items
The disciplines of big data are emerging out of a complex interplay of physical, political, technological, regulatory, and institutional factors. Firms should identify specific big data patterns that they want to operate in the cloud now. At the top of the list are big data departmental apps that (1) exploit data already in the cloud and (2) can exploit more cohesive big data stacks from cloud-based suppliers of tools. Avoid the temptation to assume that all big data apps will end up in the cloud. The costs of data movement are real and permanent and will always impact big data architectures.
Methodology
Wikibon developed its big data in the public cloud market projections using an overarching “Top Down, Bottom Up” research methodology. The base is established using a bottom-up methodology, looking at the detailed results of big data vendors and their participation in the public cloud. We also drew on the prior four years of Wikibon research in the big data and cloud markets. This research was based on:
- Scores of interviews with big data vendors in early 2016 focusing on public cloud and other topics
- Extensive user surveys in the Spring of 2014 and the Fall of 2015 http://wikibon.com/wikibon-big-data-analytics-survey-fall-2015/ to explore the major issues, concerns, and trends in the big data market – including public cloud use
- SiliconAngle/Wikibon Cube panels and interviews at big data events such as Spark Summit East
- Company public revenue figures, earnings reports, and demographics
- Media reports
- Venture capital and resellers information
- Feedback from the SiliconAngle/Wikibon community of IT practitioners
- Prior Wikibon Public Cloud research http://wikibon.com/public-cloud-market-forecast-2015-2026
For our “Top-Down” analysis, Wikibon Big Data, Cloud, and Infrastructure analysts considered the current state and potential of a technology in the context of capability and the potential business value that is deliverable and create overall adoption scenarios. We leavened that with both supply-side (vendor revenue and directions, product segment conditions) and demand-side (user deployment, expectations, application benefits, adoption friction and business attitudes) perspectives.
We believe a ten-year forecast window is preferable to a five year forecast for emerging and dynamic markets because we feel there are significant market forces – both providers and users – that won’t play out completely over a shorter time period. By extending our window we are able to better describe these trends and how Wikibon believes they will play out.
In prior forecasts, Wikibon treated Public Cloud as a separate revenue category – including allocating software delivered on public clouds by 3rd party software vendors as public cloud revenue (and not software). In this year’s big data report http://wikibon.com/2016-2026-worldwide-big-data-market-forecast/ , we simply counted big data software delivered in the public cloud as software. This would also include native big data offerings from public cloud providers like AWS, Microsoft, and Google.
In terms of handling 3rd party software license billings on the part of public cloud providers such as AWS, Microsoft Azure, and Google, the revenue was attributed to the software provider. As such we are treating this revenue as a pass-through from the cloud provider to the software provider. We feel this better captures the market activity in terms of characterizing market share.
Essentially, public cloud services represent an alternative delivery channel for big data products and services. As such it is an orthogonal view of the big data market and will overlap that data, i.e., the revenue between the two reports is not mutually exclusive and collectively exhaustive.