Contributing Analysts:
Ralph Finos
Peter Burris
David Floyer
George Gilbert
Executive Summary
The big data market grew 23.5% in 2015, led by Hadoop platform revenues. We believe the market will grow from $18.3B in 2014 to $92.2B in 2026 – a strong 14.4% CAGR (Figure 1). Growth throughout the next decade will take place in three successive and overlapping waves of application patterns – Data Lakes, Intelligent Systems of Engagement, and Self-Tuning Systems of Intelligence. Increasing amounts of data generated by sensors from the Internet of Things will drive each application pattern. Big data tool integration, administrative simplicity, and developer adoption are keys to growth rates. The adoption of streaming technologies, which address a number of Hadoop limitations, also will be a factor. Ultimately, the market growth will depend on enterprises: Will doers take the steps required to transform business with big data systems?
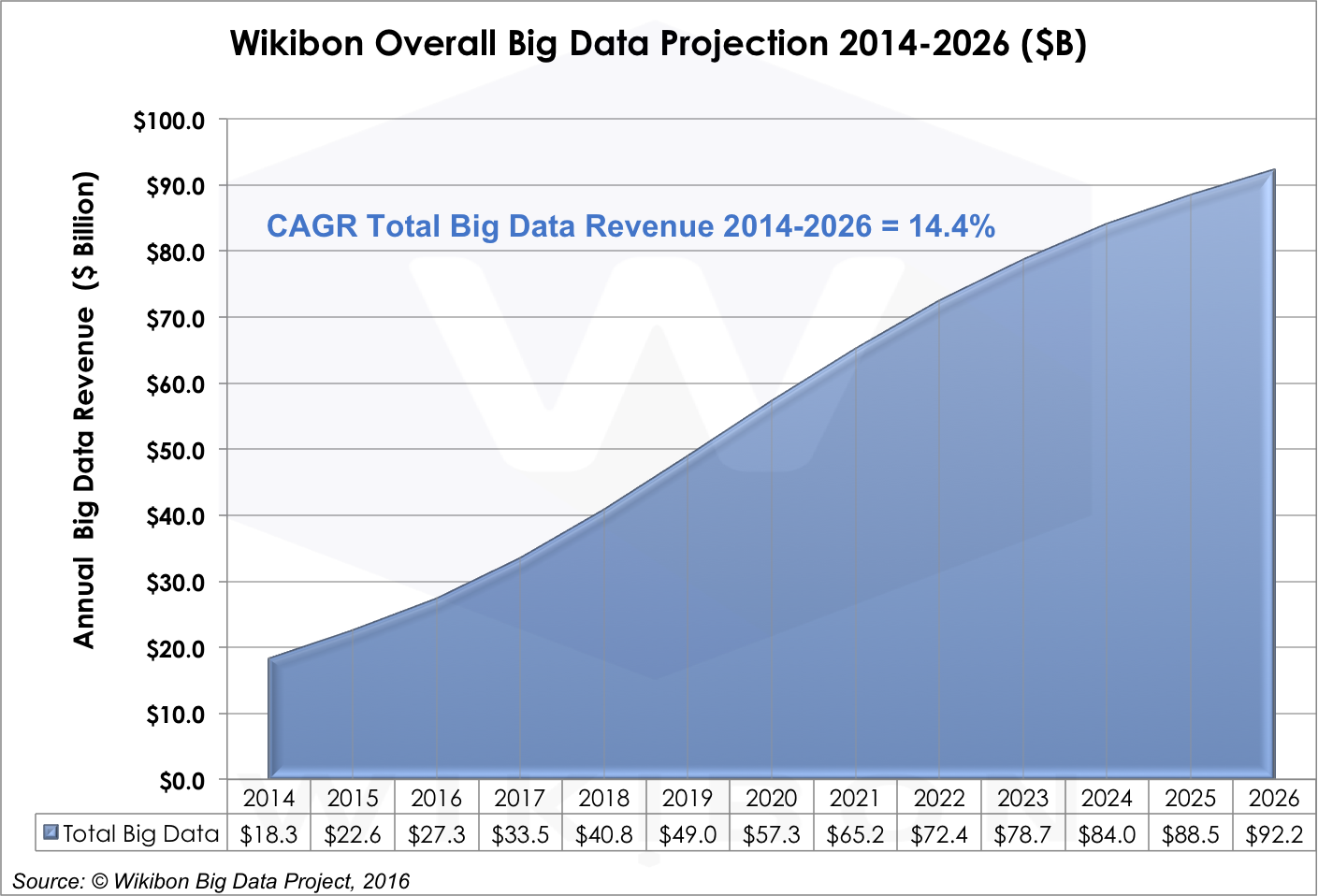
Source: © Wikibon 2016-2026 Worldwide Big Data Market Forecast
Overview
Wikibon’s 2016 Worldwide Big Data Market Forecast report is our 4th annual big data forecasts. In this 2016 report, we have adjusted our definitions to better reflect the growing market maturity of big data, and define clearer boundaries between big data and general analytics (See Wikibon Big Data Definitions and Methodology for details). The focus of this report is on patterns of application usage, drivers and barriers, and how these developments will interact with the products and services that will enable big data through 2026.
This report joins two related Wikibon forecast reports that cover additional views of this developing market. The first forecasts the big data market by application pattern. The second forecasts the evolution of streaming technologies, especially Spark (LINK HERE) These two reports should be read together with this one to understand the usage patterns and technology trends that are fueling the explosive growth we’re seeing in the forecast period.
2015-16 Big Data Market Situation and Near Term Outlook
2015 was a breakthrough year for big data. And if there is one thing Wikibon can predict, it is that 2016 will be more of the same. This was the first year that big data became a budget line item for many users. Demand for big data technology fueled Hortonworks’ explosive growth and the rapid uptake in Spark and related streaming technologies. Wikibon’s Big Data Analytics Survey Fall 2015 http://wikibon.com/wikibon-big-data-analytics-survey-fall-2015/ indicated the seriousness of big data decision makers: twice as many respondents were using purchased Hadoop distributions than was the case in our Spring 2014 survey. Enterprises are moving beyond the exploratory and discovery stage of innovation into real investment in innovation.
Our survey also indicated that among enterprises who had deployed big data solutions, 20% of the solutions were in production and 80% were proof-of-concept (PoC). Wikibon expects many PoCs will scale to production in 2016, which will catalyze new spending across all big data categories.
The big data market today is concentrated in Global 1000 enterprises and emerging Web-based, data-driven companies. Large enterprises seek to be more data driven and are investing heavily in related big data technologies and services. Cloud-native Web-based companies, like Uber, Netflix, and AirBnB, are born with big data at their core. We expect that to remain the case for the next few years, especially in the area of web-based customer and operational analytics.
Wikibon believes that the US is far in the lead today. Wikibon expects slower uptake internationally and among SMBs until the adoption barriers are reduced over time and with experience. Public cloud looks to be an appealing solution for many SMBs, although latency will be a challenge for some.
Forecast Assumptions: 2017 and Beyond
Like all emerging markets, the market for big data technologies experiences breakthroughs and encounters new obstacles almost daily. Generally, though, big data breakthroughs overcome obstacles which ensures healthy market growth for years to come. Growth drivers include Spark, public cloud, and investment by the traditional analytics heavyweights (IBM, Oracle, SAP, etc.) from the supply side. From the demand side comes the promise of the significant business benefits of IoT, huge predictive business process pay-offs (e.g., health care, finance, predictive process operations), and the opportunity to embed these algorithms into real-time business process operations.
Drivers of Big Data Adoption
Demand-Side Growth Drivers
Big data use cases are as diverse as the businesses that use big data. Enterprises are using big data to model operations, engage customers, increase portfolio returns, reduce fraud — the list goes on and on. However, despite this diversity of uses, the big data market is starting to coalesce around three accretive application patterns, which will build on each other in successive waves over the next 5-8 years.
- Maturing Data Lakes. Most enterprises today are investing in standalone HDFS repositories. This the “data lake application pattern,” which is rapidly maturing and will peak as the focus of the market by 2020. There will continue to be significant value for this use case and the related analytics, but we think demand for this use case will drop below double digital growth by 2021 as enterprises turn attention from repository to machine learning technologies. Nonetheless, Data Lakes is projected to represent 39% of the Big Data market by 2026.
- Evolving Intelligent Systems of Engagement. Integrating big data capabilities into other application domains is the top “to-do” at many advanced adopting enterprises. Simplifying the technology base and reducing latency across design and run-time tool chains is crucial. The use case possibilities are myriad, but anticipating, predicting, and ultimately influencing customer-facing digital experiences are driving the adoption of the “intelligent systems of engagement” big data pattern. Wikibon believes that Intelligent Systems of Engagement will grow significantly to 23% of the big data Market by 2026.
- Emerging Intelligent Self-Tuning Systems – An even more significant opportunity is to use machine learning to continually improve predictive accuracy. Prediction and anticipation is even more compelling when it is embedded in high-speed business processes where human real-time intervention is impossible. Wikibon projects that Intelligent Self-Tuning Systems will begin to accelerate rapidly towards the end of the decade, and become as prominent (38%) as Data Lakes by 2026.
Supply Side Growth Drivers
Big data technologies can have significant impacts on revenue-facing functions like marketing, sales, product management, services, and fulfillment. Also, it promises new approaches to optimizing cost-facing functions like finance, supply chain, and manufacturing. In other words, big data is tied to big potential spending, and the supply-side of the market is working feverishly to advance the technology:
- Increasing administrator and developer productivity– A non-integrated approach to develop is likely to lead to a disparate tool set, and that’s what has happened with Hadoop. A host of established vendors and start-ups are working the problems of improved data preparation and analysis tool chains and streamlined analytic data pipelines. Spark has been enthusiastically received and our forecast is for strong growth due to its versatility as a micro-batch, interactive, and streaming tool. Its suitability for streaming applications is key for the development of foundation technology for the emerging Intelligent Systems of Engagement and Self-Tuning Systems where the full potential of big data technology will be realized.
- Public Cloud – Public cloud will become more of a factor in big data analytics – especially for web-based enterprises, medium to large businesses, and as an important adjunct to the big data strategies of large-to-very large enterprises. There are concerns about lock-in and user inexperience with handling public cloud assets efficiently in big data use cases, but utilizing the considerable assets of public cloud providers for scaling from PoC to production, sandbox and application development, and access to unique, infrequently-used assets and tools make it an attractive option for many use cases.
- Industry Leadership – IBM, SAP, Oracle, SAS, and other leaders in the business analytics market are increasing their investment and skills to adopt and implement big data technologies and expertise required to help their customers become more effective. IBM’s embrace of Spark is one example of industry leaders stepping forward forcefully to embrace better solutions to problems that are holding back a critical market opportunity. This sort of vendor endorsement and leadership is a key factor for introducing business-altering data and analytics into critical business processes.
Adjacent Technology Growth Drivers
Big data technologies are enabling invention in other data-rich technology domains. Two in particular stand out and will co-evolve with advances in big data:
- The Internet of Things (IoT). IoT – especially industrial sensor-based – promises to enable capital-intensive enterprises to better manage, operate, and optimize their expensive assets. Utilities and oil and gas field extractors can better manage and optimize the performance of their complex systems by drawing on their assets in a more finely calibrated and efficient manner. Manufacturers of capital equipment can use IoT technology to predict and ameliorate threatening equipment conditions and repair or adjust performance remotely or in the field. In addition, Big data analytics can help manufacturers design better and more failure-resistant products, improving product quality and profitability. Edge systems will be a significant distributed portion of Big Data, as most of the data will be relevant only at the distributed site. For many applications, it will be quicker and cheaper to send a query to the edge machine and send just the results of the query back to the cloud.
- Rich Media – Mining and analyzing video, images, and other rich media is a significant opportunity in health care, surveillance, and the entertainment industries.
Obstacles to Big Data Adoption
Big data represents an inflection point not just for analytics, but the entire technology industry — and business overall. Prior to big data, being a “customer-driven” business was more of an aspirational value than an operational goal. Big data also is a technology business model experiment. It’s the first technology domain built using an open source approach. While the overall analytics market features traditional business model and development approaches to creating tooling, Hadoop and its tool sets are open source from the ground up, and from supplier to customer. While there has been significant growth in big data in the past 2-3 years, there are obstacles that will continue to be a drag on growth until they are overcome.
The Big Data Software Ecosystem Is Still Evolving
Big data technology today is still immature, which makes the successful deployment of potentially high value business workloads more expensive and risky for early adopters. The big data solution stack has many open source components and low levels of integration and support. The big data platforms today for Data Lakes — Hadoop and its complementary data preparation and analysis tool chain — has to undergo major transformation to expand its presence outside the early adopter community.
- Simplification will increase success rates. Big data investments are hit or miss because the extensive array of tools is complex, most being spawned by different open source projects that don’t necessarily share common objectives or visions. Spark and related technologies seek to address this diversity, but design and run-time analytic chains still require significant bespoke integration investments.
- Application packaging isn’t settled. The lack of application packages for big data-oriented workloads is also a drag on the market. But the problem isn’t just the lack of packages, it’s also the lack of technologies and conventions for packaging big data-related intellectual property into software packages. The good news is that the market is flooded with venture-funded start-ups attacking the data preparation and management problem from various angles – as well as the more traditional analytics and data warehouse providers who are adapting products specifically for the unique characteristics of big data. But Wikibon believes that we are a year or two away from moving beyond the major tuning and special handling that big data requires today.
- Emerging solutions demand non-batch processing. Today’s Hadoop-based implementations operate mainly in batch mode, which constraints turn-around times and limits opportunities for deeper integration of big data results in operational applications. Spark technologies are an important attempt to address that limit. Wikibon expects that Spark and related technologies will evolve to streamline pipeline and integration latencies, opening up an even more valuable vein of big data solutions.
Vendors and Users Are Building Big Data Competencies
Wikibon surveys of big data practitioners have shown that the skills gap is the #1 barrier to big data application deployments for many enterprises. The mismatch between demand for and supply of big data scientists will remain a significant drag on the market through the remainder of this decade. Alleviating the gap will require both more skilled people and accelerated delivery of standards and conventions for big data programming models, administration processes, and insight-delivery methods. Moreover, the application of big data solutions to business processes and operations is an additional challenge for enterprises beyond just the analytics tools and related math. In the end, analytics is all about optimization, prediction, explanation, and anticipation of next events. Enterprise business analysts and users need to determine the what, when, and how of the outcomes of big data analytics that best turn the math into reliable discovery and automated business value.
Data Governance Is Problematic
In addition to the challenges of managing data velocity, volume, and variety, the sources of big data are going to come from locations both inside and outside the enterprise. The successful first generation big data deployments today often involve clickstream and adtech which come from Internet Cloud sources. However, enterprises with global deployments such as finance, healthcare, and government, have concerns about security, privacy, application control, data control, and compliance. Moreover, some high value application pipelines may cross enterprises – i.e., supply chains involving manufacturers, shippers, distributors, and retailers – which will present its own set of management and governance problems. Technology can only partially solve these problems and best enterprise practice in big data governance is not likely to evolve until the 2nd half of the forecast period 2020 and beyond.
The Big Data Market Forecast – Software, Hardware & Professional Services Projections
Revenues in the 2015 worldwide big data market are split among Professional Services at 40%, Hardware at 31%, and Software at 29% (see Figure 2). Our 2026 projection shows a very different split: software will be the focus of 46% of spending, followed by professional services at 29% and hardware at 25% . Why the dramatic shift? As the market codifies big data conventions and algorithms diffuse, the market can create higher quality software and reduce the need for services (see Figure 3).
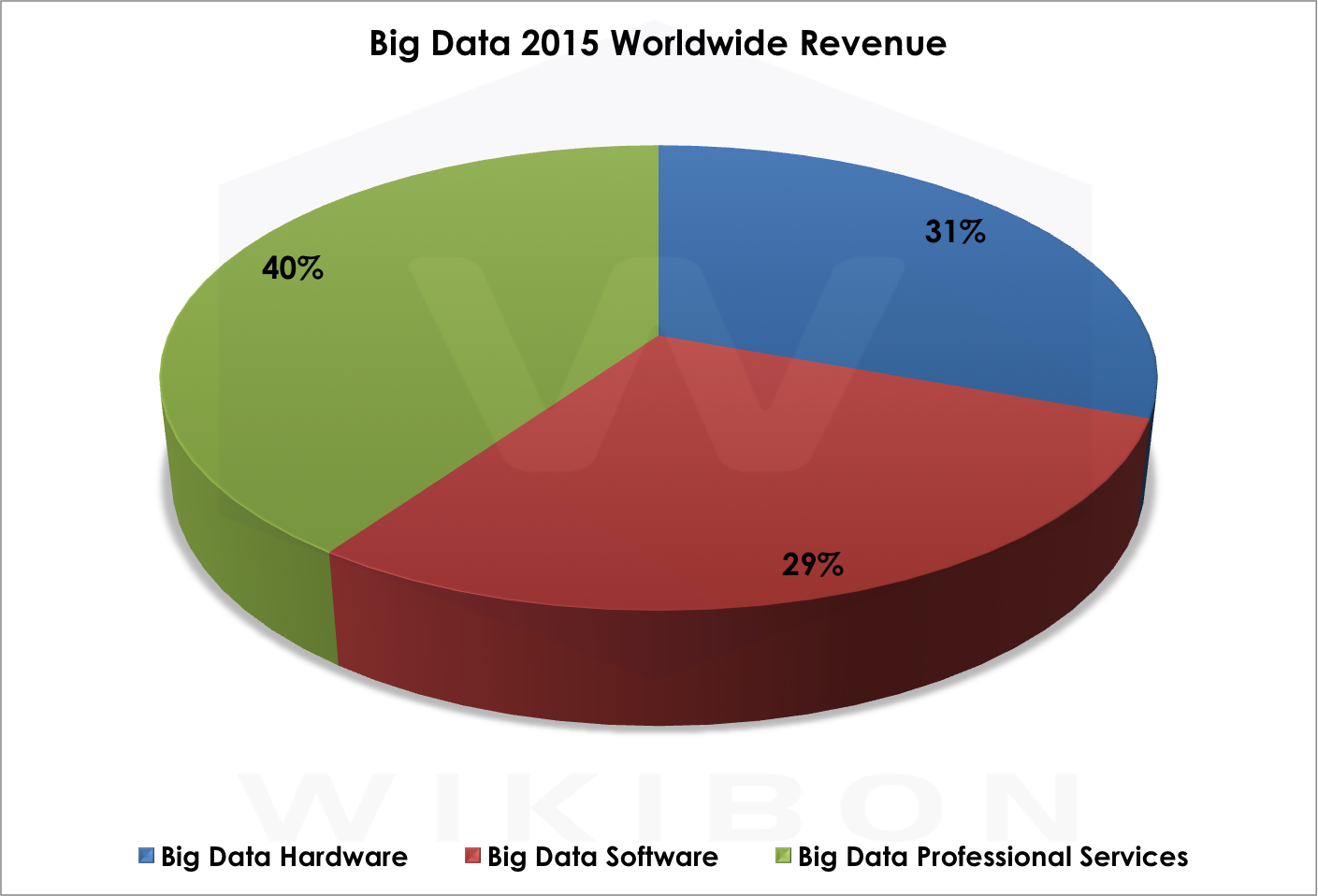
Source: Wikibon Big Data Project, 2016
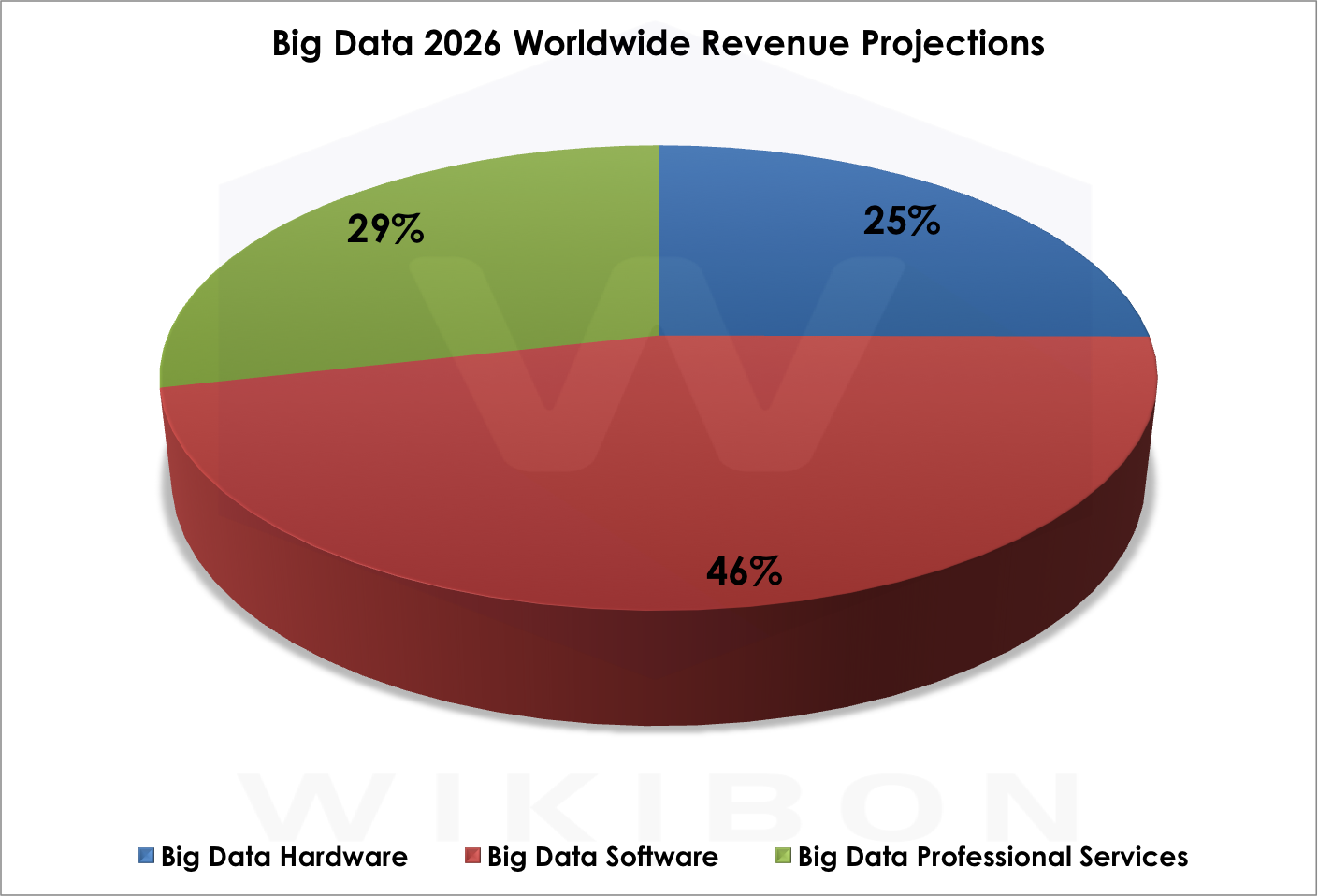
Source: Wikibon Big Data Project, 2016
Figure 4 below shows the growth trajectory of hardware, software, and services revenue in the big data market. The dynamics behind the splits and growth rates are simple: Today’s complex collection of tooling, which requires significant integration services to work, converges, which catalyzes development of increasingly sophisticated big data business software and reduces the need for integration services.
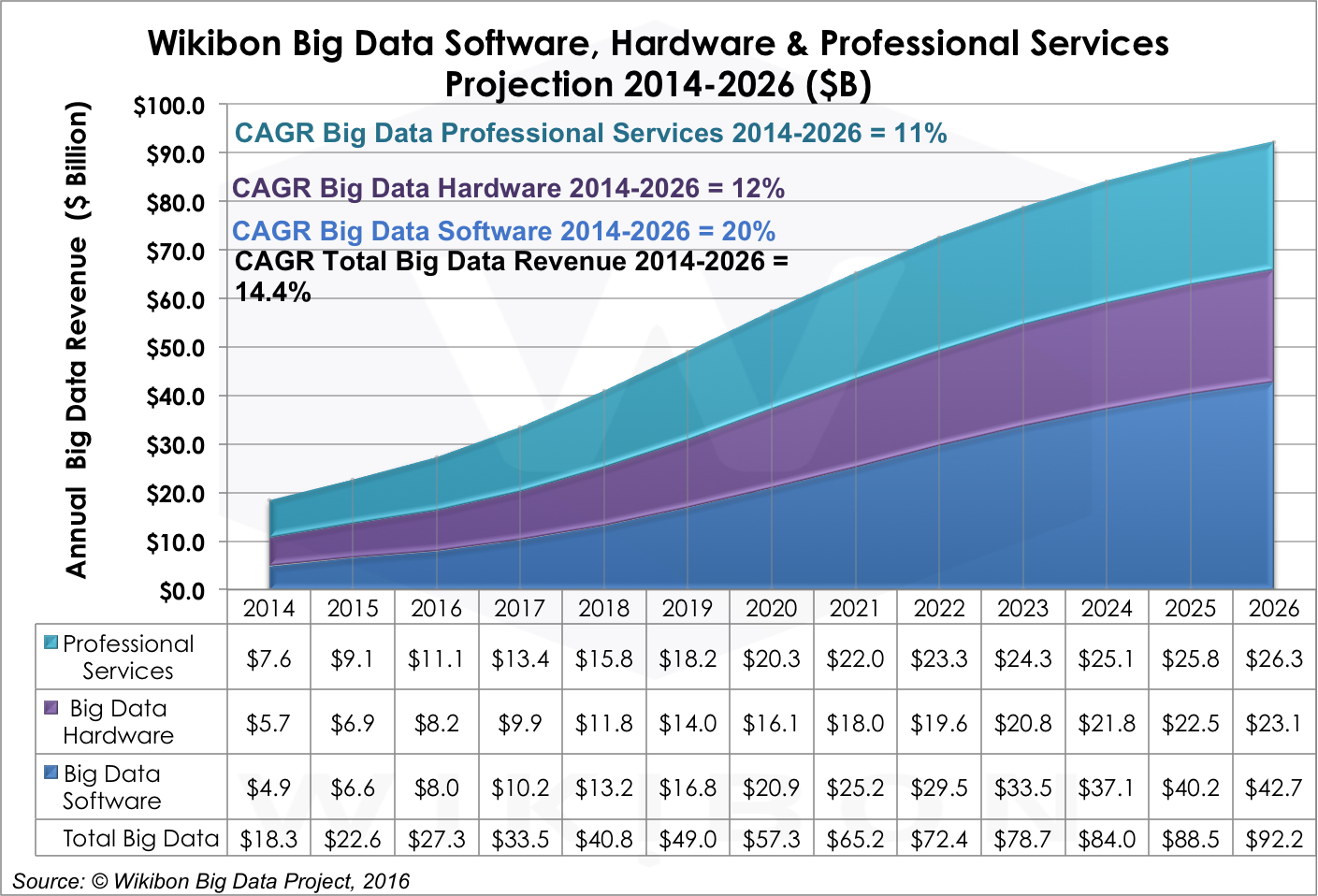
Source: Wikibon Big Data Project, 2016
Big Data Hardware Projections by Compute, Storage & Network
Big data workloads are driving many hardware design and development decisions in today’s market. The needs of big data are specific enough that the biggest big data shops, like Google, actually design, assemble, and operate their own compute, storage, and networking elements. While this may be right for Google and other Original Design Manufacturers (ODMs), most big data workloads will run on components sourced from hardware manufacturers, which will drive aggregate big data hardware revenues to $23.1B by 2026.
The arrangement of hardware spending, however, will reflect the needs of big data workloads, including (1) store more data, (2) move larger volumes of data faster through a system, (3) accelerate processing of increasingly complex data formats, and (4) automate as much stack administration as possible — even as laws and conventions for data governance and compliance rapidly evolve.
Enterprise architects will be challenged to ensure the right data is available from the right source for the right workload – as well as determining what data is expendable when. Smart meter data, for instance, feeds maintenance, billing, marketing, strategic planning, load balancing, power grid performance and many other functions at a utility. Drawing, staging, and filtering the right data for the right job is no small task.
Throughout our forecast period, big data workloads will continue to drive the leading edge of hardware performance, similar to the role that high performance computing plays in science and engineering and low latency trading in finance. Performance will be Job #1 throughout the entire forecast period and will not abate in the out years as streaming workloads become increasingly real-time.
Moreover, it should be recognized that the value of data declines rapidly with age. Wikibon believes that unless the data is used in real-time, or has known value down-stream, it should not be kept. The data from the sensors in a brake system in a car can be used instantaneously to optimize braking, but keeping the data would only be switched on if there was a specific problem to be solved. Sensible data reduction will be built into IoT applications at the edge, and only data streams that offer specific value will be saved. However, this process will not be 100% efficient, and more data will be saved than processed.
Big data Hardware (12% CAGR 2014-2026) will scale out in size as the market moves from PoC to production applications and scales up in CPU and memory and storage to meet the demands of Intelligent Systems – especially Self-Tuning ones. Public cloud IaaS will provide a counter-weight to hardware spending by continuing to bringing compute, storage, and networking costs down for PoC and more modest use cases. HP, Dell, IBM, Oracle, EMC, NetApp, Cisco and ODM offerings are the leaders in the hardware segment.
Figure 5 displays our big data hardware forecasts, which state that:
- Compute will grow 11% through 2026. Big data tooling like Hadoop is enabled by cheap, high-powered compute clusters; the two technologies are symbiotic and are co-evolving. Despite this connection, compute investments will be slower than other segments in large part because ODMs build their own compute modules and don’t buy from traditional suppliers of compute products, which will constrain revenue growth.
- Storage will grow 13% CAGR through 2026. The big data market for storage will grow as three factors evolve. First, the number of data sources will explode as IoT and other technologies mature, which will generate more data to be captured. Second, more complex data formats often require that more data be stored. Third, data science practices often encourage retaining data for longer periods, for use in evaluating past and future models, and in providing audit and compliance data. As a result of these factors, the storage market is expected to grow faster that the overall hardware market. Our forecast call for big data storage spending to eclipse big data compute spending in the 2024 time frame, and continue to grow to $11B by 2026.
- Networking will grow 16% CAGR through 2026. Networking is a key component of the big data hardware market. However, the nature of big data tasks are compute and storage, so networking is a less crucial factor here today. Over the long, however, as big data hybrid clouds become a more frequent mode of operation, networking will take on greater importance, both between locations and within the data center.
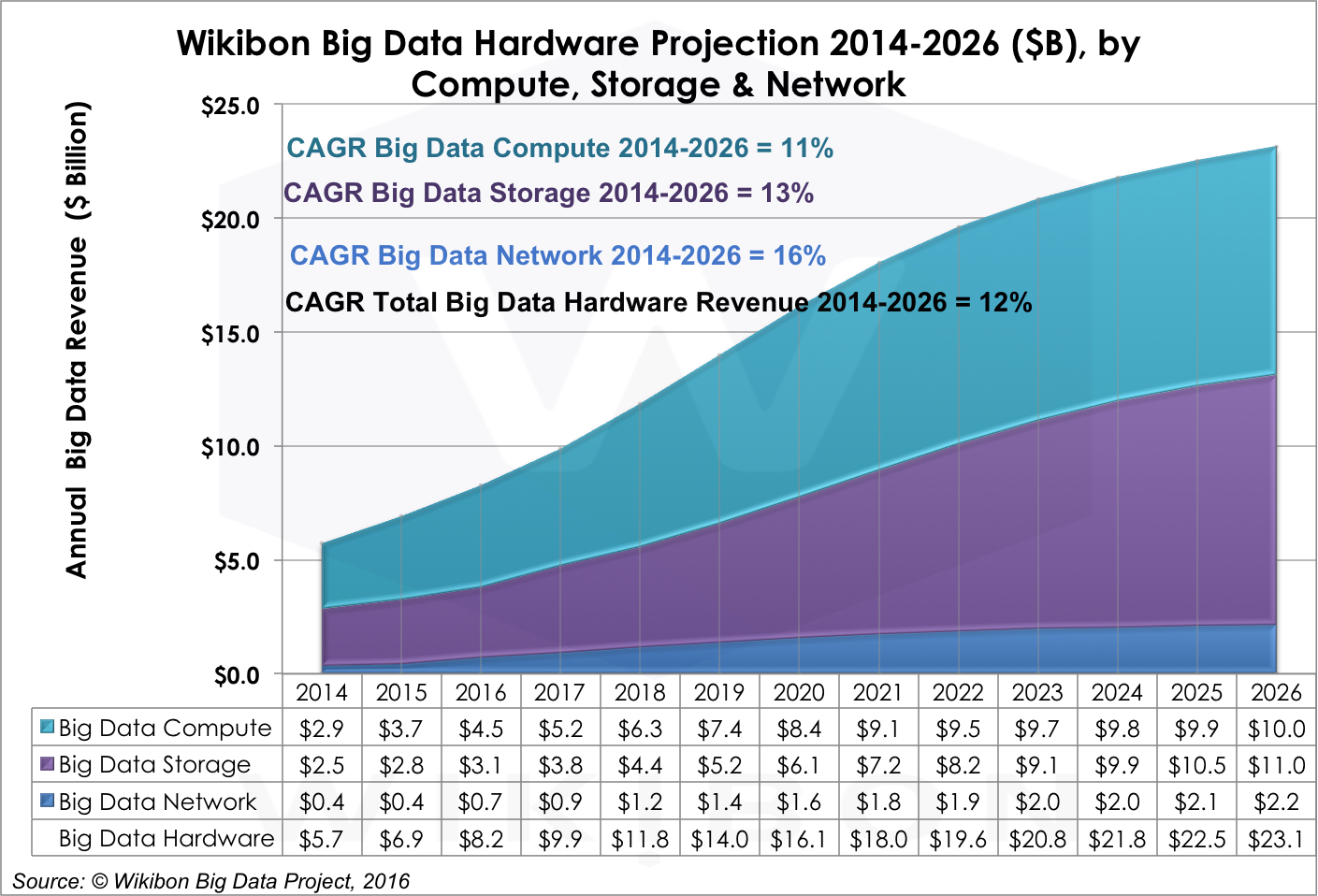
Source: © Wikibon Big Data Project, 2016
Big Data Software Projections by Data Management, Core Technologies, Database and Applications/Analytics/Tools
The market for big data software is comprised of four fundamental segments: (1) core technologies; (2) data management; (3) database management; and (4) applications, analytics, and tools software. Change in each segment affects the performance of the other segments. Why? Two reasons. First, in our forecast period, the drive to simplify tooling and packaging will cause multiple categories to converge. Second, advances in one category make possible advances in another. For example, advancing the quality of application development tooling in the data management category will spur changes in the big data applications category.
As shown in Figure 4 above, big data software is projected to grow at a CAGR of 20% from 2014-2026, significantly faster than hardware and professional services. Figure 6 below shows how that spending breaks down by software type:
- Data Management revenue will grow at a 14% CAGR through 2026. Data Management is a crucial segment in the big data market, but will experience significant restructuring over the next few years as tools start a natural process of converging. Data management includes tools for data integration, data transformation for analysis, applications development, data veracity and quality management, data governance and compliance, and process integration. Provider examples include IBM, SAP, Informatica, Oracle, Talend, Syncsort, Datameer, Attunity, Paxata Trifacta Tibco, DataTorrent, Attivio, and Altiscale. During the 2016-2021 period we expect slightly higher growth (16% CAGR). Wikibon expects these critical components for supporting efficient and effective analytics to make it easier to create more sophisticated big data workloads. Indeed, this category is a gate on overall big data market progress, especially tooling for application development. The venture community is seeding this capability which will begin to have a significant impact on Big Data adoption in this timeframe.
- Core Technologies (Hadoop, Spark, & Streaming) will grow 24% CAGR through 2026. Core technologies such as Hadoop, Spark, streaming and other big data foundation software had explosive growth in 2015 and are projected to grow at an aggressive rate through 2026. Examples of providers of Hadoop Platforms include Cloudera, Hortonworks, MapR, IBM, AWS, Pivotal and Teradata. Tools in this segment also will converge, but revenue growth will remain robust as complementary technologies are developed to solve increasingly sophisticated problems. Spark and streaming software are key to this process. Near-term, Spark reduces the latency of data movement; longer-term, it provides greater integration of advanced function, simplifying design and run-time tool chains. Wikibon expects vendors to find rational approaches to monetize these technologies, most of which are open source, including successfully packaging integrated tool sets to support a truly functioning big data ecosystem that can provide the infrastructure platform to enable effective application deployments.
- Big Data Database will grow 18% CAGR through 2026. During our forecast period, we expect traditional software vendors will adapt their relational database tool sets to capture big data workloads. The advantage of this approach is that tool migration is costly. However, big data workloads are evolving rapidly, creating demand for database technologies capable of processing new types of data faster, more cheaply, and more reliably. Given the significant paybacks of big data, we believe that multiple types of database engine will co-exist. Big data traditional software tools would include offerings from IBM, HP, SAP, Oracle, Pivotal, and Teradata. Our analysis also now includes vendors such as AWS, MarkLogic, DataStax, MongoDB, Couchbase, and Basho. We expect spending to remain very strong in the 2016-2021 period (24% CAGR) as these tools will be central to many big data solutions. In the 2022-2026 period (7% CAGR), however, we expect Database to become a more commoditized component of most every analytic and data-related task or repository.
- Big Data Applications, Analytics, and Tools Software will grow 23% through 2026. This category includes Applications, Analytics, Visualization, and Query tools from vendors like IBM, SAP, Palantir, SAS Institute, Splunk, Tableau Software, and Qlik. Fraud detection and recommendation engine platforms are examples of what’s available today. It will grow from $2.0 billion in 2014 to $23.5 billion in 2026, a CAGR of 23%. The evolution of this category is especially sensitive to the rate of convergence in other big data software categories. Why? Because as the productivity of other elements of the big data stack increases, more talent can be devoted to creating concrete big data applications. Professional services firms are crucial to creating bespoke software for enterprises today, but our expectation is the marketplace will find ways to generalize experience with client-specific applications to create application packages that can be used by broad market segments. In the meantime, the requirement for analytics, visualization of outcomes, and query tools will grow as business analysts and users want to gain access to big data analytic outcomes. Also, enterprise must pay close attention to the systems employed to diffuse insights generated by big data applications, ensuring that insights are both created and can be acted upon. The evolution of machine learning technologies will be crucial in this segment because it will drive a new class of big data capabilities and applications. Figure 7 shows the significant growth in big data Applications vs. Analytics & Tools during the forecast period ($9.4B in 2026, a 37% CAGR).
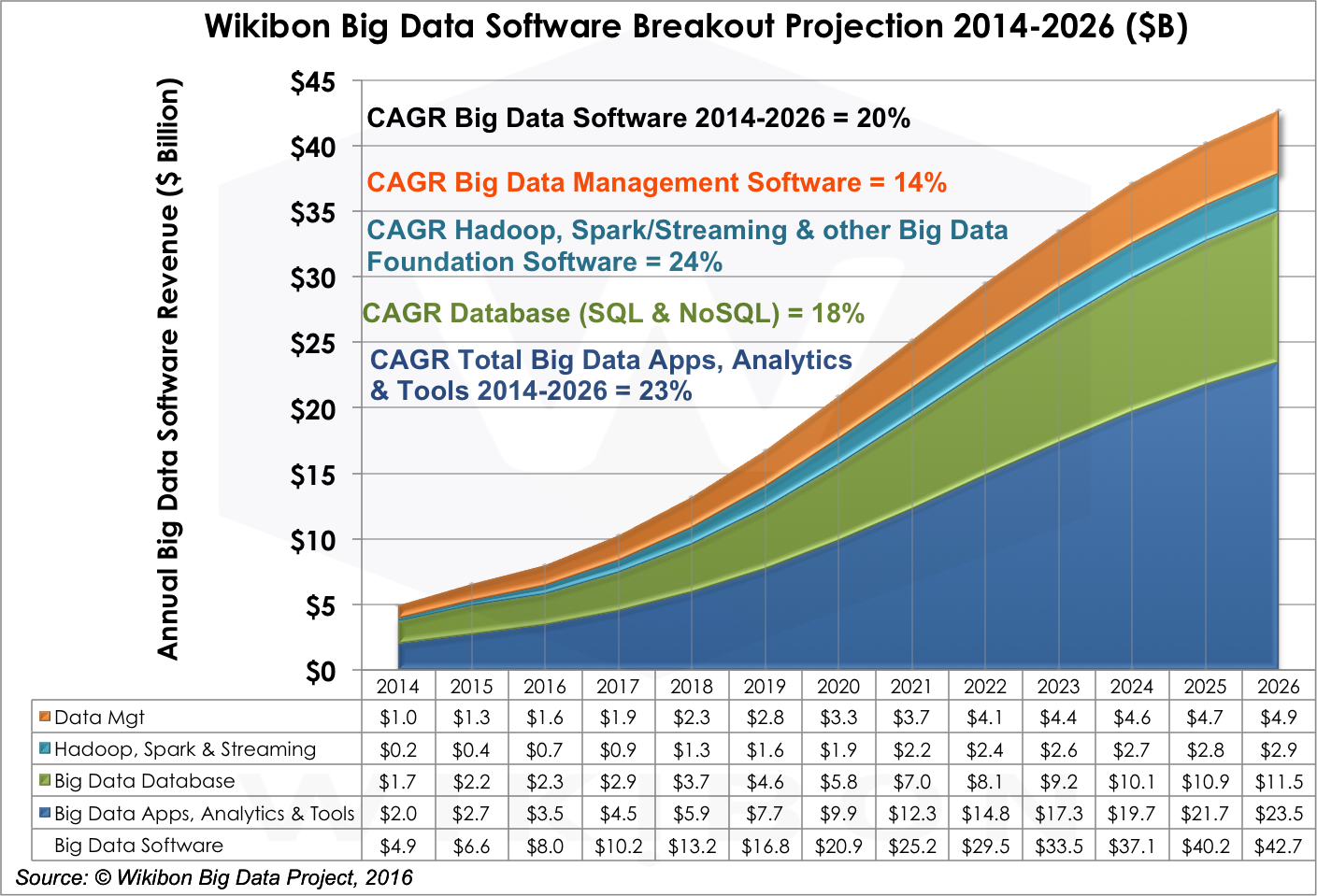
Source: © Wikibon 2016-2026 Worldwide Big Data Market Forecast
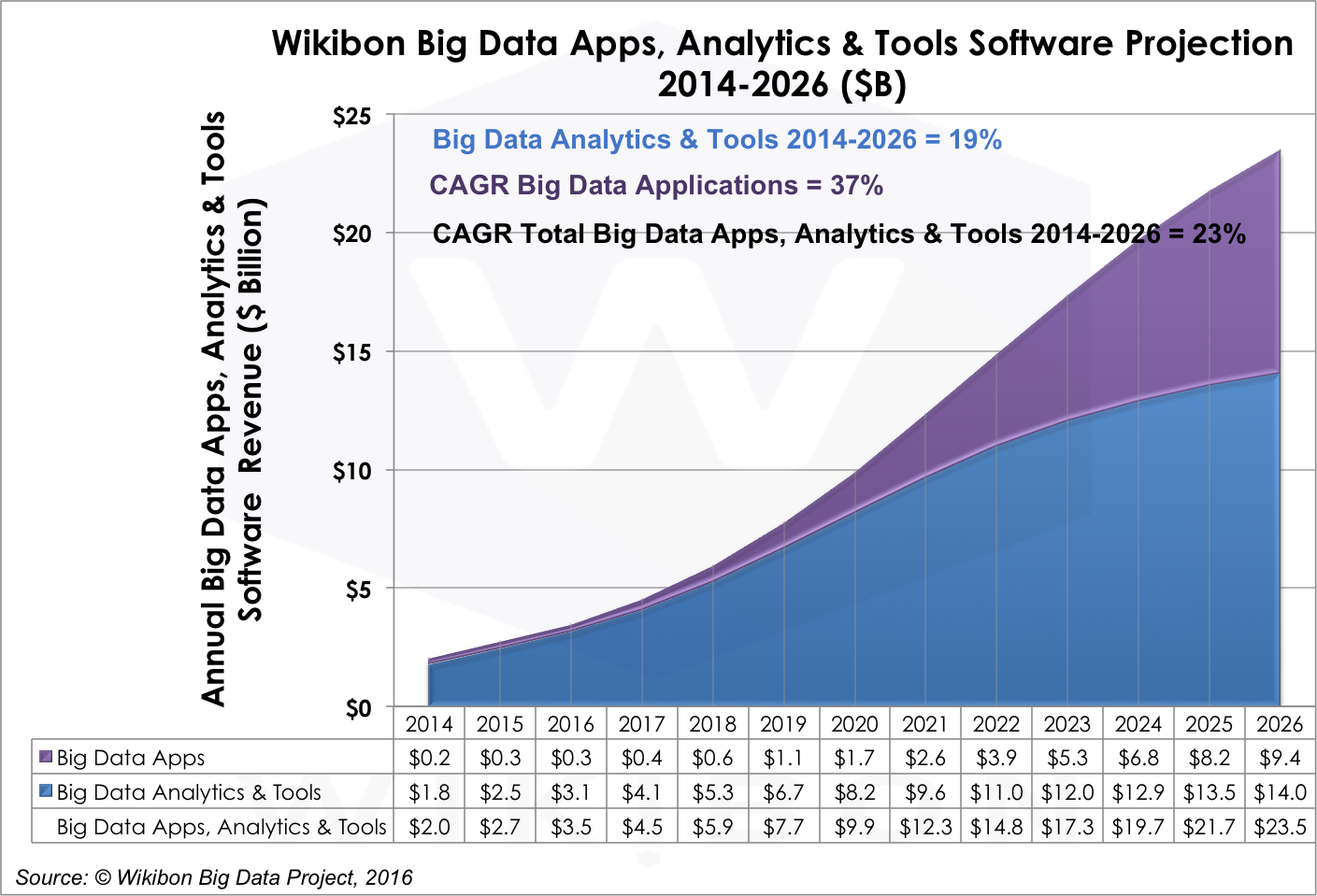
Source: © Wikibon 2016-2026 Worldwide Big Data Market Forecast
Big Data Professional Services Projections
The relationship between big data business software and big data professional services is complex, but will follow a pretty simple dynamic: As the ecosystem gains more experience with big data tools, methods, and conventions, that experience will translate into new and higher quality applications, reducing the need for lower level, integration-type professional services.
Today, professional services is the largest segment of the big data market today and will remain so through 2021, growing at an 11% CAGR (See figure 8). Professional services firms will continue to fill in the big data skills gap that exists in many enterprises.
However, new technologies, like machine learning and real-time analytics, will continue to push the technology envelope, creating new sources of demand for professional services throughout the big data stack through at least 2022. Today, IBM, Accenture, CSC, and Palantir are examples of the leaders in big data Professional Services, but this is a rich market opportunity for skilled consultants: lots of new entrants can be expected.
Our forecast says that the professional services market will slow to a 3% CAGR from 2022-2026 because we believe that cloud options will become increasingly viable for an expanding portion of the big data software stack. As that happens, professional services firms will be forced to chase increasingly esoteric opportunities, primarily vertical business processes.
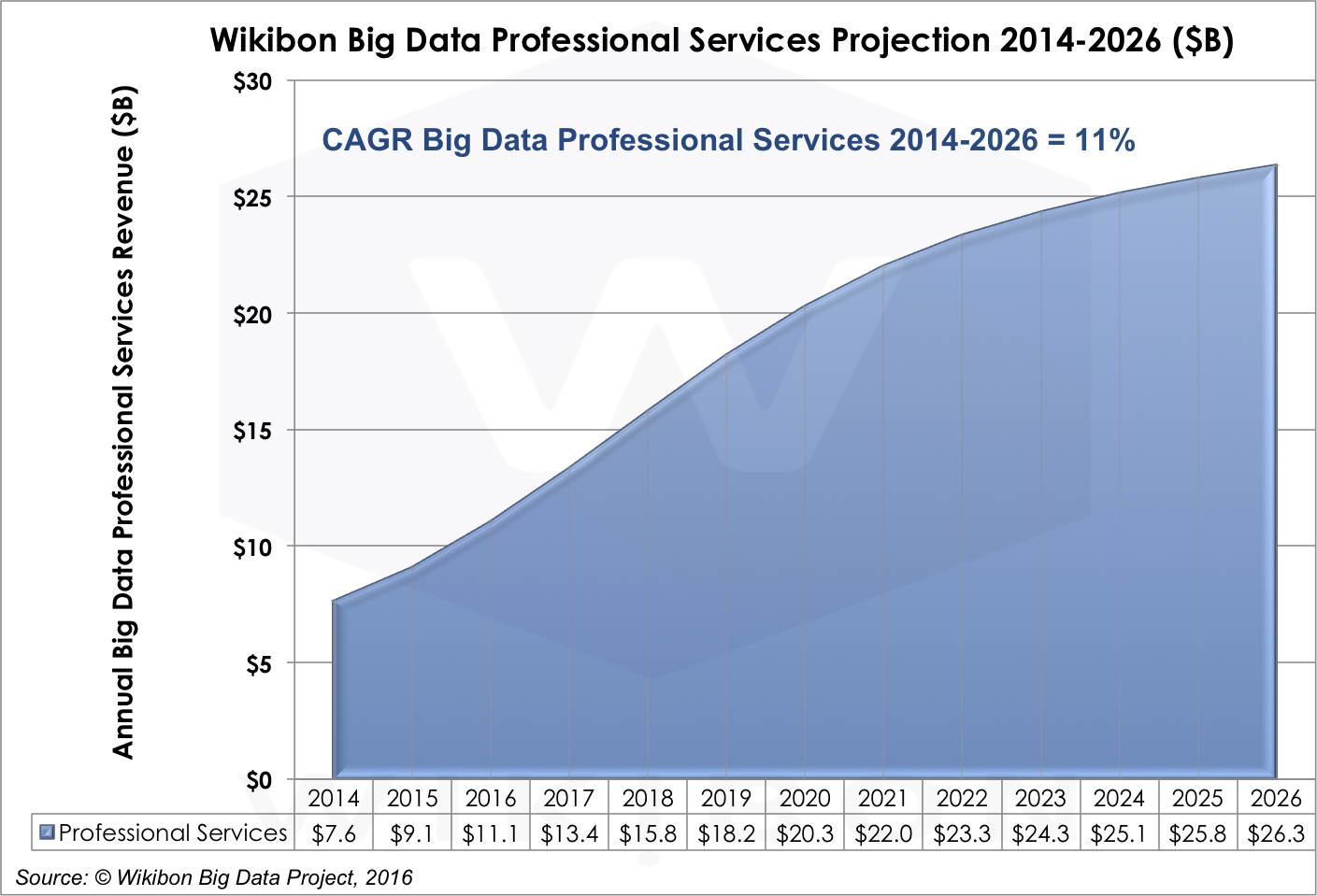
Source: © Wikibon 2016-2026 Worldwide Big Data Market Forecast
Conclusions/Advice/Summary
Enterprises, users, and technology vendors need to embrace the potential of big data analytics. Enterprises need to invest in this technology to discover how it can be applied to their business processes. While they should expect to start small with proofs of concept they should plan to invest in developing skills, gaining experience, figuring out how to apply this technology to their high value workloads, and determining which partners can help them get to the next steps.
Management – It is cautionary to look at the adoption of data warehouse technologies. Most enterprise have been disappointed in the returns of investment from data warehousing investments. Enterprises underestimated the extent that insights would be shared and acted upon.
Wikibon would strongly recommend less emphasis be placed on making a few people smarter. Wikibon recommends setting clear project success goals that include automating business processes. This can be achieved by setting the goal of integrating real-time big data processing into the systems of engagement and systems of intelligence. To succeed, close cooperation and integration is required between big data scientists, analysts from the lines of business and analysts from current IT systems. Creating organizational structures that facilitate this will be a strong predictor of success.
Doers – business analysts, app developers, and business users – need to educate themselves with the potential for this technology and where it can fit into their analytic tasks today as well as discover where it can make a substantial difference tomorrow. Get as much hands on experience as practicable and seek out opportunities to become part of early big data project opportunities.
Vendors need to develop a big data software ecosystem that can help enterprises realize the potential of big data, thereby making big data an efficient and effective marketplace.
Methodology
Wikibon develop its disruptive market projections using an overarching “Top down, Bottom up” research methodology. The base is established using a bottom-up methodology, looking at the detailed results of all the Big Data vendors. For the last four years, Wikibon has looked in depth at Big Data market size for the current year, and looked back at previous years to confirm or adjust previous figures. and forecast for this and related reports was determined based on extensive research involving:
- Scores of interviews with Big Data vendors
- Two extensive surveys in the Spring of 2014 and the Fall of 2015 to explore the major issues, concerns, and trends in the Big Data market
- SiliconAngle/Wikibon Cube panels and interviews at Big Data events such as Spark Summit East
- Company public revenue figures, earnings reports, and demographics
- Media reports
- Venture capital and resellers information
- Feedback from the SiliconAngle/Wikibon community of IT practitioners
Wikibon’s overarching research approach is “Top-Down & Bottom-Up”. That is, we consider the state and possibilities of technology in the context of potential business value that is deliverable (Top-Down) and leaven that with both supply-side (vendor revenue and directions, product segment conditions) and demand-side (user deployment, expectations, application benefits, adoption friction and business attitudes) perspectives. We believe a ten-year forecast window is preferable to a five year forecast for emerging and dynamic markets because we feel there are significant market forces – both providers and users – that won’t play out completely over a shorter time period. By extending our window we are able to better describe these trends and how Wikibon believes they will play out.
Treatment of Public Cloud
In prior years, Wikibon segmented out Public Cloud as a separate revenue category – including lifting software delivered on public clouds by 3rd party software vendors. We believe that this software should be simply counted in our big data software. This would also include native big data offerings from public cloud providers like AWS and Google.
In its essence, public cloud services represent an alternative delivery channel for big data products and services. As such it is an orthogonal view of the market and will be covered in an upcoming Wikibon report.
Treatment of SQL vs. NoSQL Segments
In prior years, Wikibon segmented database into two separate markets – SQL and NoSQL. In this report we have merged them into a single big data database category. While this was a useful distinction in prior years, database technology is evolving to accommodate traditional and alternative data models under a single umbrella where multiple data models can be handled within a single database framework.